










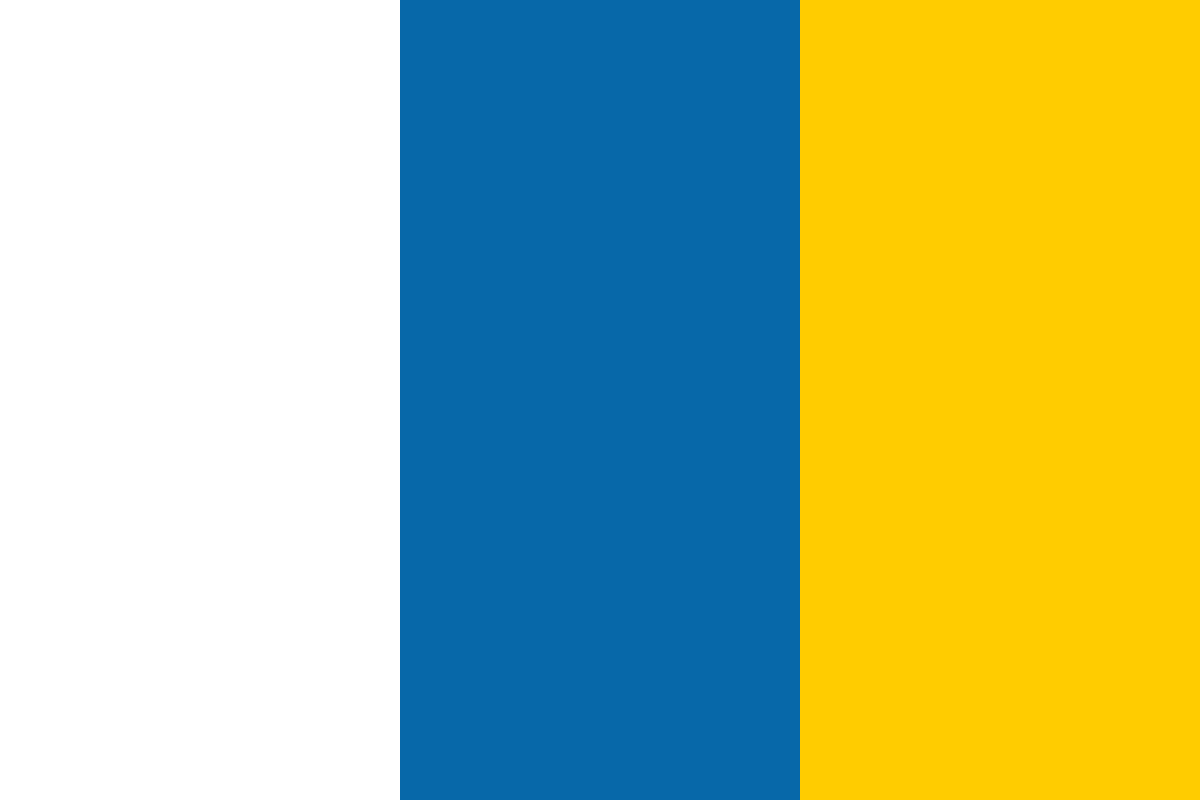

































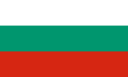








In the last decade, we saw a significant shift in how modern, internet-scale applications are being built. Cloud computing (infrastructure as a service) and containerization technologies (popularized by Docker) enabled a new breed of distributed system designs commonly referred to as microservices (and their next incarnation, FaaS). Successful companies like Twitter and Netflix have been able to leverage them to build highly scalable, efficient, and reliable systems, and to deliver more features faster to their customers.
In this article we explain the concept of observability in microservices, its challenges and traditional monitoring tools in microservices.
This article is an extract taken from the book Mastering Distributed Tracing, written by Yuri Shkuro. This book will equip you to operate and enhance your own tracing infrastructure. Through practical exercises and code examples, you will learn how end-to-end tracing can be used as a powerful application performance management and comprehension tool.
While there is no official definition of microservices, a certain consensus has evolved over time in the industry. Martin Fowler, the author of many books on software design, argues that microservices architectures exhibit the following common characteristics:
Because of the large number of microservices involved in building modern applications, rapid provisioning, rapid deployment via decentralized continuous delivery, strict DevOps practices, and holistic service monitoring are necessary to effectively develop, maintain, and operate such applications. The infrastructure requirements imposed by the microservices architectures spawned a whole new area of development of infrastructure platforms and tools for managing these complex cloud-native applications. In 2015, the Cloud Native Computing Foundation (CNCF) was created as a vendor-neutral home for many emerging open source projects in this area, such as Kubernetes, Prometheus, Linkerd, and so on, with a mission to "make cloud-native computing ubiquitous."
Read more on Honeycomb CEO Charity Majors discusses observability and dealing with “the coming armageddon of complexity” [Interview]
The term "observability" in control theory states that the system is observable if the internal states of the system and, accordingly, its behavior, can be determined by only looking at its inputs and outputs. At the 2018 Observability Practitioners Summit, Bryan Cantrill, the CTO of Joyent and one of the creators of the tool dtrace, argued that this definition is not practical to apply to software systems because they are so complex that we can never know their complete internal state, and therefore the control theory's binary measure of observability is always zero (I highly recommend watching his talk on YouTube: https://youtu.be/U4E0QxzswQc). Instead, a more useful definition of observability for a software system is its "capability to allow a human to ask and answer questions". The more questions we can ask and answer about the system, the more observable it is.
Figure 1: The Twitter debate
There are also many debates and Twitter zingers about the difference between monitoring and observability. Traditionally, the term monitoring was used to describe metrics collection and alerting. Sometimes it is used more generally to include other tools, such as "using distributed tracing to monitor distributed transactions." The definition by Oxford dictionaries of the verb "monitor" is "to observe and check the progress or quality of (something) over a period of time; keep under systematic review."
However, it is better scoped to describing the process of observing certain a priori defined performance indicators of our software system, such as those measuring an impact on the end-user experience, like latency or error counts, and using their values to alert us when these signals indicate an abnormal behavior of the system. Metrics, logs, and traces can all be used as a means to extract those signals from the application. We can then reserve the term "observability" for situations when we have a human operator proactively asking questions that were not predefined. As Bryan Cantrill put it in his talk, this process is debugging, and we need to "use our brains when debugging." Monitoring does not require a human operator; it can and should be fully automated.
"If you want to talk about (metrics, logs, and traces) as pillars of observability–great.
The human is the foundation of observability!" -- BryanCantrill
In the end, the so-called "three pillars of observability" (metrics, logs, and traces) are just tools, or more precisely, different ways of extracting sensor data from the applications. Even with metrics, the modern time series solutions like Prometheus, InfluxDB, or Uber's M3 are capable of capturing the time series with many labels, such as which host emitted a particular value of a counter. Not all labels may be useful for monitoring, since a single misbehaving service instance in a cluster of thousands does not warrant an alert that wakes up an engineer. But when we are investigating an outage and trying to narrow down the scope of the problem, the labels can be very useful as observability signals.
By adopting microservices architectures, organizations are expecting to reap many benefits, from better scalability of components to higher developer productivity. There are many books, articles, and blog posts written on this topic, so I will not go into that. Despite the benefits and eager adoption by companies large and small, microservices come with their own challenges and complexity. Companies like Twitter and Netflix were successful in adopting microservices because they found efficient ways of managing that complexity. Vijay Gill, Senior VP of Engineering at Databricks, goes as far as saying that the only good reason to adopt microservices is to be able to scale your engineering organization and to "ship the org chart".
So, what are the challenges of this design? There are quite a few:
When we see that some requests to our system are failing or slow, we want our observability tools to tell us the story about what happens to that request.
Traditional monitoring tools were designed for monolith systems, observing the health and behavior of a single application instance. They may be able to tell us a story about that single instance, but they know almost nothing about the distributed transaction that passed through it. These tools "lack the context" of the request.
It goes like this: "Once upon a time…something bad happened. The end." How do you like this story? This is what the chart in Figure 2 tells us. It's not completely useless; we do see a spike and we could define an alert to fire when this happens. But can we explain or troubleshoot the problem?
Figure 2: A graph of two time series representing (hypothetically) the volume of traffic to a service
Metrics, or stats, are numerical measures recorded by the application, such as counters, gauges, or timers. Metrics are very cheap to collect, since numeric values can be easily aggregated to reduce the overhead of transmitting that data to the monitoring system. They are also fairly accurate, which is why they are very useful for the actual monitoring (as the dictionary defines it) and alerting.
Yet the same capacity for aggregation is what makes metrics ill-suited for explaining the pathological behavior of the application. By aggregating data, we are throwing away all the context we had about the individual transactions.
Logging is an even more basic observability tool than metrics. Every programmer learns their first programming language by writing a program that prints (that is, logs) "Hello, World!" Similar to metrics, logs struggle with microservices because each log stream only tells us about a single instance of a service. However, the evolving programming paradigms creates other problems for logs as a debugging tool. Ben Sigelman, who built Google's distributed tracing system Dapper, explained it in his KubeCon 2016 keynote talk as four types of concurrency (Figure 3):
Figure 3: Evolution of concurrency
Years ago, applications like early versions of Apache HTTP Server handled concurrency by forking child processes and having each process handle a single request at a time. Logs collected from that single process could do a good job of describing what happened inside the application.
Then came multi-threaded applications and basic concurrency. A single request would typically be executed by a single thread sequentially, so as long as we included the thread name in the logs and filtered by that name, we could still get a reasonably accurate picture of the request execution.
Then came asynchronous concurrency, with asynchronous and actor-based programming, executor pools, futures, promises, and event-loop-based frameworks. The execution of a single request may start on one thread, then continue on another, then finish on the third. In the case of event loop systems like Node.js, all requests are processed on a single thread but when the execution tries to make an I/O, it is put in a wait state and when the I/O is done, the execution resumes after waiting its turn in the queue.
Both of these asynchronous concurrency models result in each thread switching between multiple different requests that are all in flight. Observing the behavior of such a system from the logs is very difficult, unless we annotate all logs with some kind of unique id representing the request rather than the thread, a technique that actually gets us close to how distributed tracing works.
Finally, microservices introduced what we can call "distributed concurrency." Not only can the execution of a single request jump between threads, but it can also jump between processes, when one microservice makes a network call to another. Trying to troubleshoot request execution from such logs is like debugging without a stack trace: we get small pieces, but no big picture.
In order to reconstruct the flight of the request from the many log streams, we need powerful logs aggregation technology and a distributed context propagation capability to tag all those logs in different processes with a unique request id that we can use to stitch those requests together. We might as well be using the real distributed tracing infrastructure at this point! Yet even after tagging the logs with a unique request id, we still cannot assemble them into an accurate sequence, because the timestamps from different servers are generally not comparable due to clock skews.
In this article we looked at the concept of observability and some challenges one has to face in microservices. We further discussed traditional monitoring tools for microservices.
Applying distributed tracing to microservices-based architectures will be easy with Mastering Distributed Tracing written by Yuri Shkuro.
6 Ways to blow up your Microservices!
Have Microservices killed the monolithic architecture? Maybe not!
How to build Dockers with microservices