










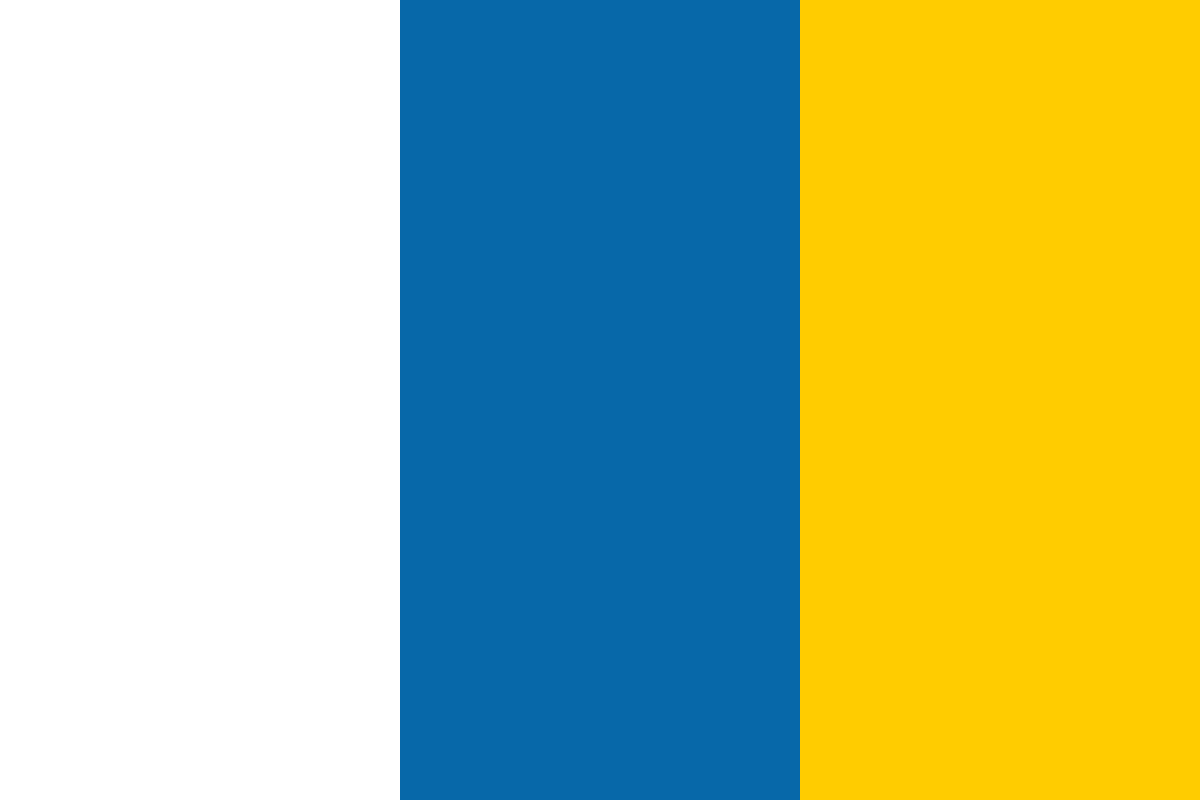

































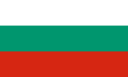








I recently stumbled across an old Uber blog that offended me. It was a puerile clickbait article that claimed to be able to identify the areas in a city that were more prone to engage in one-night stands. It also offended me in its assumptions about the data it presented to support its claim. This blog isn’t about the group of people that it calls “RoGers” (“Ride of Glory-ers”), it’s about how when you go looking for results, you can find whatever data you need. If you want to read it in its full glory, the original Uber article can be found here.
So. Some facts. People who have one night stands (according to Uber):
From the A–B locations and a somewhat artificial timeframe, we’re lead to believe that we’re looking at the sinners of San Francisco. Let’s have a look at an alternate reality where there are fewer people having Uber-fuelled sex.
My theory is this; young people go out. They go out after the hours of 10pm, and return before 6am. They go out to drink within a few miles of their home. They do this, as all good employees, on a Friday and Saturday night because, well, who likes working with a hangover? I will call them ReGs (Regular Everyman Guys/Girls).
To establish where people actually live, I took a sample from 91,000 apartment listings from datasf and ran it through Google Map Engine, which lets n00bs like me create maps:
Google Map Engine only lets me do 500 rows of data for free, so it’s limited, but you can see that most people live in north-eastern San Francisco. This will come as no surprise to people who live there, but as I’ve only ever seen San Francisco in films (Homeward Bound et al.), I thought it prudent to prove it. Basically, we can establish that people live where Uber say they live. Lock the doors, this is going to be a wild one.
I think the maximum age of decency for a 6-hour drinking bender is probably about 33. So I needed to know that a large portion of the RoGer area was full of young people occupying these apartments. Finding an age map of a city was really difficult, but after Googling “San Franciso Age Map” I found one at http://synthpopviewer.rti.org/. The blue represents ages 15–34. Red is 55-64. Young people live in San Francisco! Who knew?
More specifically, the “heat map” areas seem to match up nicely to the Uber data:
A city full of young people. What do they do at night? Are they really RoGers?
There’s an article from growthhackers that says the no.1 reason for Uber use (and subsequent) growth is “Restaurants and Nightlife”. It seems like a reasonable assumption that people want to drink rather than drive, so I mapped out the restaurants in San Fran (hoping that restaurants = bars and clubs too). Again, there’s a clear grouping around similar areas.
Young people live in San Francisco. They are surrounded by restaurants and bars. I’m using my own experiences with the body of 27,000 Birmingham students, and of being a worker in my mid-20s, that most go out on a Friday and Saturday night and that they do it after 10pm (normally about 11pm) and return at around 3am. They aren’t going out for Rides of Glory, they’re going out to practice expressive dance until the early hours.
My narrative still smells a bit right? I’m ignoring that half of the “young people” in my sample can’t drink, I’m assuming that the people who can actually go out at night, and I’m assuming that my restaurant map also represents bars and nightclubs. The data about apartment listings was basically pointless.
And the same can be said for data of the RoGers of Uber. We’re told that because a young city, full of workers and students take trips between 10pm and 6am, they’re all playing away. It’s an analysis as full of assumptions as my own. Uber knew what they wanted (more clicks) before they came to their conclusion.
When you do this in the real world, it can lead to big mistakes. Data-driven decisions aren’t a half and half approach. If you choose that path, you must be dedicated to it–get all the possible relevant data points, and allow people who know what these data points mean to come up with conclusions from them.
When you ask a question before you get the data, you end up with what you want. In this scenario, I ended up with ReGs. Uber ended up with RoGers. I think I’m more correct than they are because their conclusion is stupid. But we’re both likely to be wrong in the end. We went in to the big world of data with a question (what would make a good blog), and ended up with clouded judgment. When you’re investing the future of your company based on clouded data, this approach would have bigger implications than producing a clickbait blog. Next time, I’ll get the data first and then let that tell me what will make a good blog.