










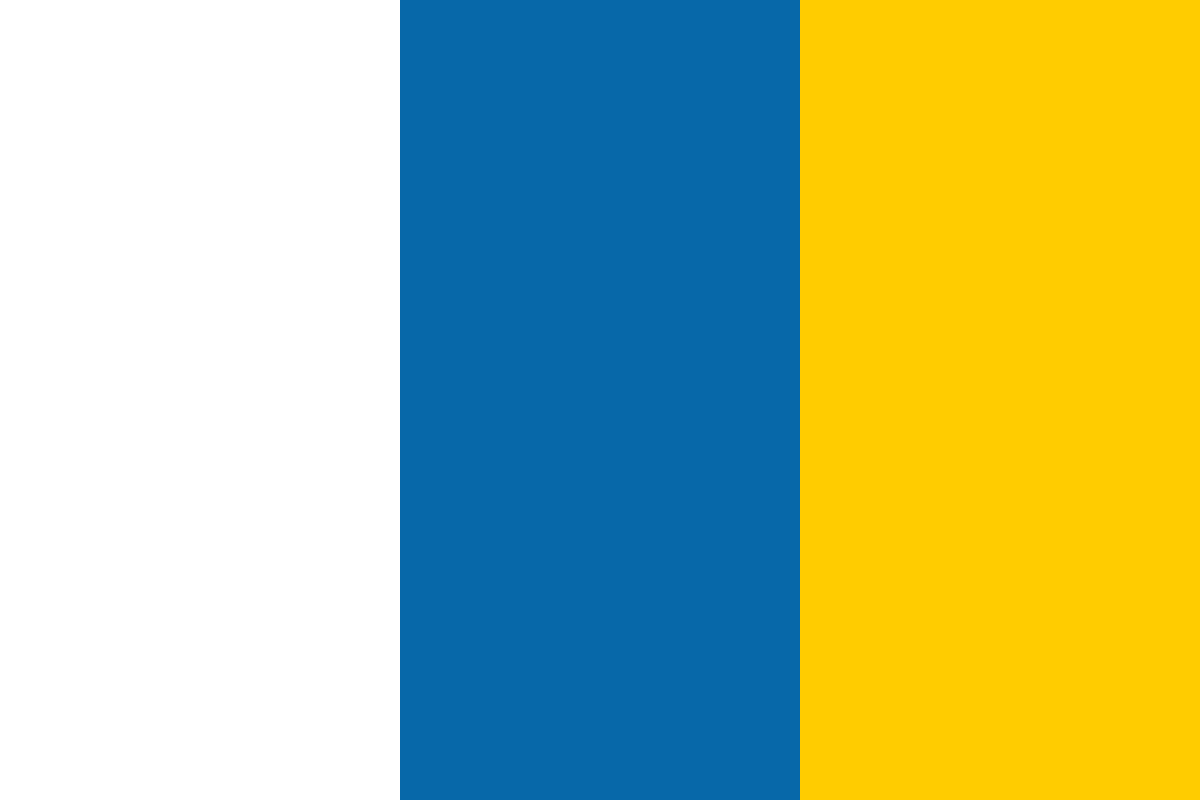

































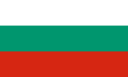








(For more resources related to this topic, see here.)
The rules surrounding technology are constantly changing. Decisions and architectures based on current technology might easily become out of date with hardware changes. To best understand how multimedia and unstructured data fit and can adapt to the changing technology, it's important to understand how and why we arrived at our different current architectural positions. In some cases we have come full circle and reinvented concepts that were in use 20 years ago. Only by learning from the lessons of the past can we see how to move forward to deal with this complex environment.
In the past 20 years a variety of architectures have come about in an attempt to satisfy some core requirements:
Allow as many users as possible to access the system
Ensure those users had good performance for accessing the data
Enable those users to perform DML (insert/update/delete) safely and securely (safely implies ability to restore data in the event of failure)
The goal of a database management system was to provide an environment where these points could be met. The first databases were not relational. They were heavily I/O focused as the computers did not have much memory and the idea of caching data was deemed to be too expensive. The servers had kilobytes and then eventually, megabytes of memory. This memory was required foremost by the programs to run in them. The most efficient architecture was to use pointers to link the data together. The architecture that emerged naturally was hierarchical and a program would navigate the hierarchy to find rows related to each other. Users connected in via a dumb terminal. This was a monitor with a keyboard that could process input and output from a basic protocol and display it on the screen. All the processing of information, including how the screen should display it (using simple escape sequence commands), was controlled in the server.
The mainframes used a block mode structure, where the user would enter a screen full of data and press the Enter key. After doing this the whole screen of information was sent to the server for processing. Other servers used asynchronous protocols, where each letter, as it was typed, was sent to the server for processing. This method was not as efficient as block mode because it required more server processing power to handle the data coming in. It did provide a friendlier interface for data entry as mistakes made could be relayed immediately back to the user. Block mode could only display errors once the screen of data was sent, processed, and returned.
As more users started using these systems, the amount of data in them began to grow and the users wanted to get more intelligence out of the data entered. Requirements for reporting appeared as well as the ability to do ad hoc querying. The databases were also very hard to maintain and enhance as the pointer structure linked everything together tightly. It was very difficult to perform maintenance and changes to code. In the 1970s the relational database concept was formulated and it was based on sound mathematical principles. In the early 1980s the first conceptual relational databases appeared in the marketplace with Oracle leading the way.
The relational databases were not received well. They performed poorly and used a huge amount of server resources. Though they achieved a stated goal of being flexible and adaptable, enabling more complex applications to be built quicker, the performance overheads of performing joins proved to be a major issue. Benefits could be seen in them, but they could never be seen as being able to be used in any environment that required tens to hundreds or thousands of concurrent users. The technology wasn't there to handle them.
To initially achieve better performance the relational database vendors focused on using a changing hardware feature and that was memory. By the late 1980s the computer servers were starting to move from 16 bit to 32 bit. The memory was increasing and there was drop in the price. By adapting to this the vendors managed to take advantage of memory and improved join performance.
The relational databases in effect achieved a balancing act between memory and disk I/O. Accessing a disk was about a thousand times slower than accessing memory. Memory was transient, meaning if there was a power failure and if there was data stored in memory, it would be lost. Memory was also measured in megabytes, but disk was measured in gigabytes. Disk was not transient and generally reliable, but still required safeguards to be put in place to protect from disk failure.
So the balancing act the databases performed involved caching data in memory that was frequently accessed, while ensuring any modifications made to that data were always stored to disk. Additionally, the database had to ensure no data was lost if a disk failed. To improve join performance the database vendors came up with their own solutions involving indexing, optimization techniques, locking, and specialized data storage structures. Databases were judged on the speed at which they could perform joins.
The flexibility and ease in which applications could be updated and modified compared to the older systems soon made the relational database become popular and must have. As all relational databases conformed to an international SQL standard, there was a perception that a customer was never locked into a propriety system and could move their data between different vendors. Though there were elements of truth to this, the reality has shown otherwise. The Oracle Database key strength was that you were not locked into the hardware and they offered the ability to move a database between a mainframe to Windows to Unix. This portability across hardware effectively broke the stranglehold a number of hardware vendors had, and opened up the competition enabling hardware vendors to focus on the physical architecture rather than the operating system within it.
In the early 1990s with the rise in popularity of the Apple Macintosh, the rules changed dramatically and the concept of a user friendly graphical environment appeared. The Graphical User Interface (GUI) screen offered a powerful interface for the user to perform data entry. Though it can be argued that data entry was not (and is still not) as fast as data entry via a dumb terminal interface, the use of colors, varying fonts, widgets, comboboxes, and a whole repository of specialized frontend data entry features made the interface easier to use and more data could be entered with less typing. Arguably, the GUI opened up the computer to users who could not type well. The interface was easier to learn and less training was needed to use the interface.
The GUI interface had one major drawback; it was expensive to run on the CPU. Some vendors experimented with running the GUI directly on the server (the Solaris operating system offered this capability), but it become obvious that this solution would not scale.
To address this, the two-tier architecture was born. This involved using the GUI, which was running on an Apple Macintosh or Microsoft Windows or other Windows environment (Microsoft Windows wasn't the only GUI to run on Intel platforms) to handle the display processing. This was achieved by moving the application displayed to the computer that the user was using. Thus splitting the GUI presentation layer and application from the database. This seemed like an ideal solution as the database could now just focus on handling and processing SQL queries and DML. It did not have to be burdened with application processing as well. As there were no agreed network protocols, a number had to be used, including named pipes, LU6.2, DECNET, and TCP/IP. The database had to handle language conversion as the data was moved between the client and the server. The client might be running on a 16-bit platform using US7ASCII as the character set, but the server might be running on 32-bit using EBCDIC as the character set. The network suddenly became very complex to manage.
What proved to be the ultimate show stopper with the architecture had nothing to do with the scalability of client or database performance, but rather something which is always neglected in any architecture, and that is the scalability of maintenance. Having an environment of a hundred users, each with their own computer accessing the server, requires a team of experts to manage those computers and ensure the software on it is correct. Application upgrades meant upgrading hundreds of computers at the same time. This was a time-consuming and manual task. Compounded by this is that if the client computer is running multiple applications, upgrading one might impact the other applications. Even applying an operating system patch could impact other applications. Users also might install their own software on their computer and impact the application running on it. A lot of time was spent supporting users and ensuring their computers were stable and could correctly communicate with the server.
Specialized software vendors tried to come to the rescue by offering the ability to lock down a client computer from being modified and allowing remote access to the computer to perform remote updates. Even then, the maintenance side proved very difficult to deal with and when the idea of a three tier architecture was pushed by vendors, it was very quickly adopted as the ideal solution to move towards because it critically addressed the maintenance issue.
In the mid 1990s the rules changed again. The Internet started to gain in popularity and the web browser was invented. The browser opened up the concept of a smart presentation layer that is very flexible and configured using a simple mark up language. The browser ran on top of the protocol called HTTP, which uses TCP/IP as the underlying network protocol.
The idea of splitting the presentation layer from the application became a reality as more applications appeared in the browser. The web browser was not an ideal platform for data entry as the HTTP protocol was stateless making it very hard to perform transactions in it. The HTTP protocol could scale. The actual usage involved the exact same concepts as block mode data entry performed on mainframe computers. In a web browser all the data is entered on the screen, and then sent in one go to the application handling the data.
The web browser also pushed the idea that the operating system the client is running on is immaterial. The web browsers were ported to Apple computers, Windows, Solaris, and Unix platforms.
The web browser also introduced the idea of standard for the presentation layer. All vendors producing a web browser had to conform to the agreed HTML standard. This ensured that anyone building an application that confirmed to HTML would be able to run on any web browser.
The web browser pushed the concept that the presentation layer had to run on any client computer (later on, any mobile device as well) irrespective of the operating system and what else was installed on it. The web browser was essentially immune from anything else running on the client computer. If all the client had to use was a browser, maintenance on the client machine would be simplified.
HTML had severe limitations and it was not designed for data entry. To address this, the Java language came about and provided the concept of an applet which could run inside the browser, be safe, and provide an interface to the user for data entry. Different vendors came up with different architectures for splitting their two tier application into a three tier one.
Oracle achieved this by taking their Oracle Forms product and moving it to the middle application tier, and providing a framework where the presentation layer would run as a Java applet inside the browser. The Java applet would communicate with a process on the application server and it would give it its own instructions for how to draw the display. When the Forms product was replaced with JDeveloper, the same concept was maintained and enhanced. The middle tier became more flexible and multiple middle application tiers could be configured enabling more concurrent users.
The three tier architecture has proven to be an ideal environment for legacy systems, giving them a new life and enabling them be put in an environment where they can scale. The three tier environment has a major flaw preventing it from truly scaling. The flaw is the bottleneck between the application layer and the database. The three tier environment also is designed for relational databases. It is not designed for multimedia databases.In the architecture if the digital objects are stored in the database, then to be delivered to the customer they need to pass through the application-database network (exaggerating the bottleneck capacity issues), and from there passed to the presentation layer.
Those building in this environment naturally lend themselves to the concept that the best location for the digital objects is the middle tier. This then leads to issues of security, backing up, management, and all the issues previously cited for why storing the digital objects in the database is ideal. The logical conclusion to this is to move the database to the middle tier to address this. In reality, the logical conclusion is to move the application tier back into the database tier.
In the mid 2000s the idea of a virtualization began to appear in the marketplace. A virtualization was not really a new idea and the concept has existed on the IBM MVS environment since the late 1980s. What made this virtualization concept powerful was that it could run Windows, Linux, Solaris, and Mac environments within them. A virtualized environment was basically the ability to run a complete operating system within another operating system. If the computer server had sufficient power and memory, it could run multiple virtualizations (VMs). We can take the snapshot of a VM, which involves taking a view of the disk and memory and storing it. It then became possible to rollback to the snapshot.
A VM could be easily cloned (copied) and backed up. VMs could also be easily transferred to different computer servers. The VM was not tied to a physical server and the same environment could be moved to new servers as their capacity increased.
A VM environment became attractive to administrators simply because they were easy to manage. Rather than running five separate servers, an administrator could have the one server with five virtualizations in it.
The VM environment entered at a critical moment in the evolution of computer servers. Prior to 2005 most computer servers had one or two CPUs in them. The advanced could have as many as 64 (for example, the Sun E10000), but generally, one or two was the simplest solution. The reason was that computer power was doubling every two years following Moore's law. By around 2005 the market began to realize that there was a limit to the speed of an individual CPU due to physical limitations in the size of the transistors in the chips. The solution was to grow the CPUs sideways and the concept of cores came about. A CPU could be broken down into multiple cores, where each one acted like a separate CPU but was contained in one chip. With the introduction of smart threading, the number of virtual cores increased. A single CPU could now simulate eight or more CPUs.
This concept has changed the rules. A server can now run with a large number of cores whereas 10 years ago it was physically limited to one or two CPUs. If a process went wild and consumed all the resources of one CPU, it impacted all users. In the multicore CPU environment, a rogue process will not impact the others. In a VM the controlling operating system (which is also called a hypervisor, and can be hardware, firmware, or software centric) can enable VMs to be constrained to certain cores as well as CPU thresholds within that core. This allows a VM to be fenced in. This concept was taken by Amazon and the concept of the cloud environment formed.
This architecture is now moving into a new path where users can now use remote desktop into their own VM on a server. The user now needs a simple laptop (resulting in the demise of the tower computer) to use remote desktop (or equivalent) into the virtualization. They then become responsible for managing their own laptop, and in the event of an issue, it can be replaced or wiped and reinstalled with a base operating system on it. This simplifies the management. As all the business data and application logic is in the VM, the administrator can now control it, easily back it up, and access it.
Though this VM cloud environment seems like a good solution to resolving the maintenance scalability issue, a spanner has been thrown in the works at the same time as VMs are becoming popular, so was the evolution of the mobile into a portable hand held device with applications running on it.
The iPhone, iPad, Android, Samsung, and other devices have caused a disruption in the marketplace as to how the relationship between the user and the application is perceived and managed.
These devices are simpler and on the face of it employ a variety of architectures including two tier and three tier. Quality control of the application is managed by having an independent and separate environment, where the user can obtain their application for the mobile device. The strict controls Apple employs for using iTunes are primarily to ensure that the Trojan code or viruses are not embedded in the application, resulting in a mobile device not requiring a complex and constantly updating anti-virus software.
Though the interface is not ideal for heavy data entry, the applications are naturally designed to be very friendly and use touch screen controls. The low cost combined with their simple interface has made them an ideal product for most people and are replacing the need for a laptop in a number of cases. Application vendors that have applications that naturally lend themselves to this environment are taking full advantage of it to provide a powerful interface for clients to use.
The result is that there are two architectures today that exist and are moving in different directions. Each one is popular and resolves certain issues. Each has different interfaces and when building and configuring a storage repository for digital objects, both these environments need to be taken into consideration.
For a multimedia environment the ideal solution to implement the application is based on the Web. This is because the web environment over the last 15 years has evolved into one which is very flexible and adaptable for dealing with the display of those objects. From the display of digital images to streaming video, the web browser (with sometimes plugins to improve the display) is ideal. This includes the display of documents.
The browser environment though is not strong for the editing of these digital objects. Adobe Photoshop, Gimp, Garage Band, Office, and a whole suite of other products are available that are designed to edit each type of digital object perfectly. This means that currently the editing of those digital objects requires a different solution to the loading, viewing and delivery of those digital objects.
There is no right solution for the tier architecture to manage digital objects. The N-Tier model moves the application and database back into the database tier. An HTTP server can also be located in this tier or for higher availability it can be located externally.
Optimal performance is achieved by locating the application as close to the database as possible. This reduces the network bottleneck. By locating the application within the database (in Oracle this is done by using PL/SQL or Java) an ideal environment is configured where there is no overhead between the application and database.
The N-Tier model also supports the concept of having the digital objects stored outside the environment and delivered using other methods. This could include a streaming server. The N-Tier model also supports the concept of transformation servers. Scalability is achieved by adding more tiers and spreading the database between them. The model also deals with the issue of the connection to the Internet becoming a bottleneck. A database server in the tier is moved to another network to help balance the load.
For Oracle this can be done using RAC to achieve a form of transparent scalability. In most situations, Tuning, scalability at the server is achieved using manual methods using a form of application partitioning.
When a database administrator first creates a database that they know will contain digital objects, they will be confronted with some basic database configuration questions covering key sizing features of the database.
When looking at the Oracle Database there are a number of physical and logical structures built inside the database. To avoid confusion with other database management systems, it's important to note that an Oracle Database is a collection of schemas, whereas in other database management the terminology for a database equates to exactly one schema. This confusion has caused a lot of issues in the past. An Oracle Database administrator will say it can take 30 minutes to an hour to create a database, whereas a SQL Server administrator will say it takes seconds to create a database. In Oracle to create a schema (the same as a SQL Server database) also takes seconds to perform.
For the physical storage of tables, the Oracle Database is composed of logical structures called tablespaces.
The tablespace is designed to provide a transparent layer between the developer creating a table and the physical disk system and to ensure the two are independent. Data in a table that resides in a tablespace can span multiple disks and disk subsystem or a network storage system. A subsystem equating to a Raid structure has been covered in greater detail at the end of this article.
A tablespace is composed of many physical datafiles. Each datafile equates to one physical file on the disk. The goal when creating a datafile is to ensure its allocation of storage is contiguous in that the operating system and doesn't split its location into different areas on the disk (Raid and NAS structures store the data in different locations based on their core structure so this rule does not apply to them). A contiguous file will result in less disk activity being performed when full tablespace scans are performed. In some cases, especially, when reading in very large images, this can improve performance.
A datafile is fragmented (when using locally managed tablespaces, the default in Oracle) into fixed size extents. Access to the extents is controlled via a bitmap which is managed in the header of the tablespace (which will reside on a datafile). An extent is based on the core Oracle block size. So if the extent is 128 KB and the database block size is 8 KB, 16 Oracle blocks will exist within the extent. An Oracle block is the smallest unit of storage within the database. Blocks are read into memory for caching, updated, and changes stored in the redo logs. Even though the Oracle block is the smallest unit of storage, as a datafile is an operating system file, based on the type of server filesystem (UNIX can be UFS and Windows can be NTFS), the unit of storage at this level can change.
The default in Windows was once 512 bytes, but with NTFS can be as high as 64 KB. This means every time a request is made to the disk to retrieve data from the filesystem it does a read to return this amount of data. So if the Oracle block's size was 8 KB in size and the filesystem block size was 64 KB, when Oracle requests a block to be read in, the filesystem will read in 64 KB, return the 8 KB requested, and reject the rest. Most filesystems cache this data to improve performance, but this example highlights how in some cases not balancing the database block size with the filesystem block size can result in wasted I/O. The actual answer to this is operating system and filesystem dependent, and it also depends on whether Oracle is doing read aheads (using the init.ora parameter db_file_multiblock_read_count).
When Oracle introduced the Exadata they put forward the idea of putting smarts into the disk layer. Rather than the database working out how best to retrieve the physical blocks of data, the database passes a request for information to the disk system. As the Exadata knows about its own disk performance, channel speed, and I/O throughput, it is in a much better position for working out the optimal method for extracting the data. It then works out the best way of retrieving it based on the request (which can be a query). In some cases it might do a full table scan because it can process the blocks faster than if it used an index. It now becomes a smart disk system rather than a dumb/blind one. This capability has changed the rules for how a database works with the underlying storage system.
In Oracle 10G, Oracle introduced ASM primarily to improve the performance of Oracle RAC (clustered systems, where multiple separate servers share the same database on the same disk). It replaces the server filesystem and can handle mirroring and load balancing of datafiles. ASM takes the filesystem and operating system out of the equation and enables the database administrator to have a different degree of control over the management of the disk system.
The database block size is the fundamental unit of storage within an Oracle Database. Though the database can support different block sizes, a tablespace is restricted to one fixed block size.
The block sizes available are 4 KB, 8 KB, 16 KB, and 32 KB (a 32 KB block size is valid only on 64-bit platforms). The current tuning mentality says it's best to have one block size for the whole database. This is based on the idea that the one block size makes it easier to manage the SGA and ensure that memory isn't wasted.
If multiple block sizes are used, the database administrator has to partition the SGA into multiple areas and assign each a block size. So if the administrator decided to have the database at 8 KB and 16 KB, they would have to set up a database startup parameter indicating the size of each:
DB_8K_CACHE_SIZE = 2G
DB_16K_CACHE_SIZE = 1G
The problem that an administrator faces is that it can be hard to judge memory usage with table usage. In the above scenario the tables residing in the 8 KB block might be accessed a lot more than 16 KB ones, meaning the memory needs to be adjusted to deal with that. This balancing act of tuning invariably results in the decision that unless exceptional situations warrant its use, it's best to keep to the same database blocks size across the whole database. This makes the job of tuning simpler.
As is always the case when dealing with unstructured data, the rules change. The current thinking is that it's more efficient to store the data in a large block size. This ensures there is less wasted overhead and fewer block reads to read in a row of data. The challenge is that the size of the unstructured data can vary dramatically. It's realistic for an image thumbnail to be under 4 KB in size. This makes it an ideal candidate to be stored in the row with the other relational data. Even if an 8 KB block size is used, the thumbnail and other relational data might happily exist in the one block. A photo might be 10 MB in size requiring a large number of blocks to be used to store it. If a 16 KB block size is used, it requires about 64 blocks to store 1 MB (assuming there is some overhead that requires overall extra storage for the block header).
An 8 KB block size requires about 130 blocks. If you have to store 10 MB, the number of blocks increases 10 times. For an 8 KB block that is over 1300 reads is sufficient for one small-sized 10 MB image. With images now coming close to 100 MB in size, this figure again increases by a factor of 10. It soon becomes obvious that a very large block size is needed. When storing video at over 4 GB in size, even a 32 KB block size seems too small.
As is covered later in the article, unstructured data stored in an Oracle blob does not have to be cached in the SGA. In fact, it's discouraged because in most situations the data is not likely to be accessed on a frequent basis. This generally holds true but there are cases, especially with video, where this does not hold true and this situation is covered later. Under the assumption that the thumbnails are accessed frequently and should be cached and the originals are accessed infrequently and should not be cached, the conclusion is that it now becomes practical to split the SGA in two. The unstructured, uncached data is stored in a tablespace using a large block size (32 KB) and the remaining data is stored in a more acceptable and reasonable 8 KB block. The SGA for the 32 KB is kept to a bare minimum as it will not be used, thus bypassing the issue of perceived wasted memory by splitting the SGA in two.
In the following table a simple test was done using three tablespace block sizes. The aim was to see if the block size would impact load and read times. The load involved reading in 67 TIF images totaling 3 GB in size. The result was that the tablespace block size made no statistical significant difference. The test was done using a 50-MB extent size and as shown shown in the next segment, this size will impact performance. So to correctly understand how important block size can be, one has to look at not only the block size but also the extent size.
Details of the environment used to perform these tests
CREATE TABLESPACE tbls_name BLOCKSIZE 4096/8192/16384 EXTENT
MANAGEMENT LOCAL UNIFORM SIZE 50M segment space management auto
datafile 'directory/datafile' size 5G reuse;
The following table compares the various block sizes:
Tablespace block size
|
Blocks
|
Extents
|
Load time
|
Read time
|
4 KB
|
819200
|
64
|
3.49 minutes
|
1.02 minutes
|
8 KB
|
403200
|
63
|
3.46 minutes
|
0.59 minutes
|
16 KB
|
201600
|
63
|
3.55 minutes
|
0.59 minutes
|
When creating a tablespace to store the unstructured data, the next step after the block size is determined is to work out what the most efficient extent size will be. As a table might contain data ranging from hundreds of gigabytes to terabytes determining the extent size is important. The larger the extent, the potential to possible waste space if the table doesn't use it all is greater. The smaller the extent size the risk is that the table will grow into tens or hundreds of thousands of extents. As a locally managed tablespace uses a bitmap to manage the access to the extents and is generally quite fast, having it manage tens of thousands of extents might be pushing its performance capabilities.
There are two methods available to the administrator when creating a tablespace. They can manually specify the fragment size using the UNIFORM extent size clause or they can let the Oracle Database calculate it using the AUTOALLOCATE clause. Tests were done to determine what the optimal fragment size was when AUTOALLOCATE was not used. The AUTOALLOCATE is a more set-and-forget method and one goal was to see if this clause was as efficient as manually setting it.
Covers testing performed to try to find an optimal extent and block size. The results showed that a block size of 16384 (16 KB) is ideal, though 8192 (8 KB) is acceptable. The block size of 32 KB was not tested. The administrator, who might be tempted to think the larger the extent size, the better the performance, would be surprised that the results show that this is not always the case and an extent size between 50 MB-200 MB is optimal.
For reads with SECUREFILES the number of extents was not a major performance factor but it was for writes.
When compared to the AUTOALLOCATE clause, it was shown there was no real performance improvement or loss when used. The testing showed that an administrator can use this clause knowing they will get a good all round result when it comes to performance. The syntax for configuration is as follows:
EXTENT MANAGEMENT LOCAL AUTOALLOCATE segment space management auto
Repeated tests showed that this configuration produced optimal read/write times without the database administrator having to worry about what the extent size should be. For a 300 GB tablespace it produced a similar number of extents as when a 50M extent size was used.
As has been covered, once an image is loaded it is rare that it is updated. A relational database fragmentation within a tablespace is caused by repeated creation/dropping of schema objects and extents of different sizes, resulting in physical storage gaps, which are not easily reused. Storage is lost. This is analogous to the Microsoft Windows environment with its disk storage. After a period of time, the disk becomes fragmented making it hard to find contiguous storage and locate similar items together. Locating all the pieces in a file as close together as possible can dramatically reduce the number of disk reads required to read it in. With NTFS (a Microsoft disk filesystem format) the system administrator can on creation determine whether extents are autoallocated or fragmented. This is similar in concept to the Oracle tablespace creation. Testing was not done to check if the fragmentation scenario is avoided with the AUTOALLOCATE clause. The database administrator should therefore be aware of the tablespace usage and whether it is likely going to be stable once rows are added (in which case AUTOALLOCATE can be used simplifying storage management). If it is volatile, the UNIFORM clause might be considered as a better option.
For working with unstructured data, the primary uses of the TEMPORARY tablespace is to hold the contents of temporary tables and temporary lobs. A temporary lob is used for processing a temporary multimedia object. In the following example, a temporary blob is created. It is not cached in memory. A multimedia image type is created and loaded into it. Information is extracted and the blob is freed. This is useful if images are stored temporarily outside the database. This is not the same case as using a bfile which Oracle Multimedia supports. The bfile is a permanent pointer to an image stored outside the database.
SQL>
declare
image ORDSYS.ORDImage;
ctx raw(4000);
begin
image := ordsys.ordimage.init();
dbms_lob.createtemporary(image.source.localdata,FALSE);
image.importfrom(ctx, 'file', 'LOADING_DIR', 'myimg.tif');
image.setProperties;
dbms_output.put_line( 'width x height = ' || image.width ||
'x' || image.height);
dbms_lob.freetemporary(image.source.localdata);
end;
/
width x height = 2809x4176
It's important when using this tablespace to ensure that all code, especially on failure, performs a dbms_lob.freetemporary function, to ensure that storage leakage doesn't occur. This will result in the tablespace continuing to grow until it runs out of room. In this case the only way to clean it up is to either stop all database processes referencing, then resize the datafile (or drop and recreate the temporary tablespace after creating another interim one), or to restart the database and mount it. The tablespace can then be resized or dropped and recreated.
The UNDO tablespace is used by the database to store sufficient information to rollback a transaction. In a database containing a lot of digital objects, the size of the database just for storage of the objects can exceed terabytes. In this situation the UNDO tablespace can be sized larger giving added opportunity for the database administrator to perform flashback recovery from user error. It's reasonable to size the UNDO tablespace at 50 GB even growing it to 100 GB in size. The larger the UNDO tablespace the further back in time the administrator can go and the greater the breathing space between user failure, user failure detected and reported, and the database administrator doing the flash back recovery.
The following is an example flashback SQL statement. The as of timestamp clause tells Oracle to find rows that match the timestamp from the current time going back so that we can have a look at a table an hour ago:
select t.vimg.source.srcname || '=' ||
dbms_lob.getlength(t.vimg.source.localdata)
from test_load as of timestamp systimestamp - (1/24) t;
The SYSTEM tablespace contains the data dictionary. In Oracle 11g R2 it also contains any compiled PL/SQL code (where PLSQL_CODE_TYPE=NATIVE). The recommended initial starting size of the tablespace should be 1500 MB.
The following test results highlight how important it is to get the size and placement of the redo logs correct. The goal was to determine what combination of database parameters and redo/undo size were optimal. In addition, an SSD was used as a comparison. Based on the result of each test, the parameters and/or storage was modified to see whether it would improve the results. When it appeared an optimal parameter/storage setting was found, it was locked in while the other parameters were tested further. This enabled multiple concurrent configurations to be tested and an optimal result to be calculated.
The test involved loading 67 images into the database. Each image varied in size between 40 to 80 MB resulting in 2.87 GB of data being loaded. As the test involved only image loading, no processing such as setting properties or extraction of metadata was performed. Archiving on the database was not enabled. All database files resided on hard disk unless specified.
In between each test a full database reboot was done. The test was run at least three times with the range of results shown as follows:
Database parameter descriptions used:
Redo Buffer Size = LOG_BUFFER
Multiblock Read Count = db_file_multiblock_read_count
Source disk
|
Redo logs
|
Database parameters
|
Fastest time
|
Slowest time
|
Hard disk
|
Hard disk
3 x 50 MB
|
Redo buffer size = 4 MB
Multiblock read count = 64
UNDO tablespace on HD (10 GB)
Table datafile on HD
|
3 minutes and 22 sec
|
3 minutes and 53 sec
|
Hard disk
|
Hard disk
3 x 1 GB
|
Redo buffer size = 4 MB
Multiblock read count = 64
UNDO tablespace on HD (10 GB)
Table datafile on HD
|
2 minutes and 49 sec
|
2 minutes and 57 sec
|
Hard disk
|
SSD
3 x 1 GB
|
Redo buffer size = 4 MB
Multiblock read count = 64
UNDO tablespace on HD (10 GB)
Table datafile on HD
|
1 minute and 30 sec
|
1 minute and 41 sec
|
Hard disk
|
SSD
3 x 1 GB
|
Redo buffer size = 64 MB
Multiblock read count = 64
UNDO tablespace on HD (10 GB)
Table datafile on HD
|
1 minute and 23 sec
|
1 minute and 48 sec
|
Hard disk
|
SSD
3 x 1 GB
|
Redo buffer size = 8 MB
Multiblock read count = 64
UNDO tablespace on HD (10 GB)
Table datafile on HD
|
1 minute and 18 sec
|
1 minute and 29 sec
|
Hard disk
|
SSD
3 x 1 GB
|
Redo buffer size = 16 MB
Multiblock read count = 64
UNDO tablespace on HD (10 GB)
Table datafile on HD
|
1 minute and 19 sec
|
1 minute and 27 sec
|
Hard disk
|
SSD
3 x 1 GB
|
Redo buffer size = 16 MB
Multiblock read count = 256
UNDO tablespace on HD (10 GB)
Table datafile on HD
|
1 minute and 27 sec
|
1 minute and 41 sec
|
Hard disk
|
SSD
3 x 1 GB
|
Redo buffer size = 8 MB
Multiblock read count = 64
UNDO tablespace = 1 GB on SSD
Table datafile on HD
|
1 minute and 21 sec
|
1 minute and 49 sec
|
SSD
|
SSD
3 x 1 GB
|
Redo buffer size = 8 MB
Multiblock read count = 64
UNDO tablespace = 1 GB on SSD
Table datafile on HD
|
53 sec
|
54 sec
|
SSD
|
SSD
3 x 1 GB
|
Redo buffer size = 8 MB
Multiblock read count = 64
UNDO tablespace = 1 GB on SSD
Table datafile on SSD
|
1 minute and 20 sec
|
1 minute and 20 sec
|
The tests show a huge improvement when the redo logs were moved to a Solid State Drive (SSD). Though the conclusion that can be drawn is this: the optimal step to perform it might be self defeating. A number of manufacturers of SSD acknowledge there are limitations with the SSD when it comes to repeated writes. The Mean Time to Failure (MTF) might be 2 million hours for reads; for writes the failure rate can be very high. Modern SSD and flash cards offer much improved wear leveling algorithms to reduce failures and make performance more consistent. No doubt improvements will continue in the future.
A redo log by its nature is constant and has heavy writes. So, moving the redo logs to the SSD might quickly result in it becoming damaged and failing. For an organization that on configuration performs one very large load of multimedia, the solution might be to initially keep the redo logs on SSD, and once the load is finished, to move the redo logs to a hard drive.
Increasing the size of the redo logs from 50 MB to 1 GB improves performance and all database containing unstructured data should have a redo log size of at least 1 GB. The number of logs should be at least 10; preferred is from 50 to 100. As is covered later, disk is cheaper today than it once was, and 100 GB of redo logs is not that large a volume of data as it once was. The redo logs should always be mirrored.
The placement or size of the UNDO tablespace makes no difference with performance.
The redo buffer size (LOG_BUFFER) showed a minor improvement when it was increased in size, but the results were inconclusive as the figures varied. A figure of LOG_BUFFER=8691712, showed the best results and database administrators might use this figure as a starting point for tuning.
The changing of multiblock read count (DB_FILE_MULTIBLOCK_READ_COUNT) from the default value of 64 to 256 showed no improvement. As the default value (in this case 64) is set by the database as optimal for the platform, the conclusion that can be drawn is that the database has set this figure to be a good size.
By moving the original images to an SSD showed another huge improvement in performance. This highlighted how the I/O bottleneck of reading from disk and the writing to disk (redo logs) is so critical for digital object loading.
The final test involved moving the datafile containing the table to the SSD. It highlighted a realistic issue that DBAs face in dealing with I/O. The disk speed and seek time might not be critical in tuning if the bottleneck is the actual time it takes to transfer the data to and from the disk to the server. In the test case the datafile was moved to the same SSD as the redo logs resulting in I/O competition. In the previous tests the datafile was on the hard disk and the database could write to the disk (separate I/O channel) and to the redo logs (separate I/O channel) without one impacting the other. Even though the SSD is a magnitude faster in performance than the disk, it quickly became swamped with calls for reads and writes. The lesson is that it's better to have multiple smaller SSDs on different I/O channels into the server than one larger channel. Sites using a SAN will soon realize that even though SAN might offer speed, unless it offers multiple I/O channels into the server, its channel to the server will quickly become the bottleneck, especially if the datafiles and the images for loading are all located on the server.
The original tuning notion of separating data fi les onto separate disks that was performed more than 15 years ago still makes sense when it comes to image loading into a multimedia database. It's important to stress that this is a tuning issue while dealing with image loading not when running the database in general. Tuning the database in general is a completely different story and might result in a completely different architecture.