










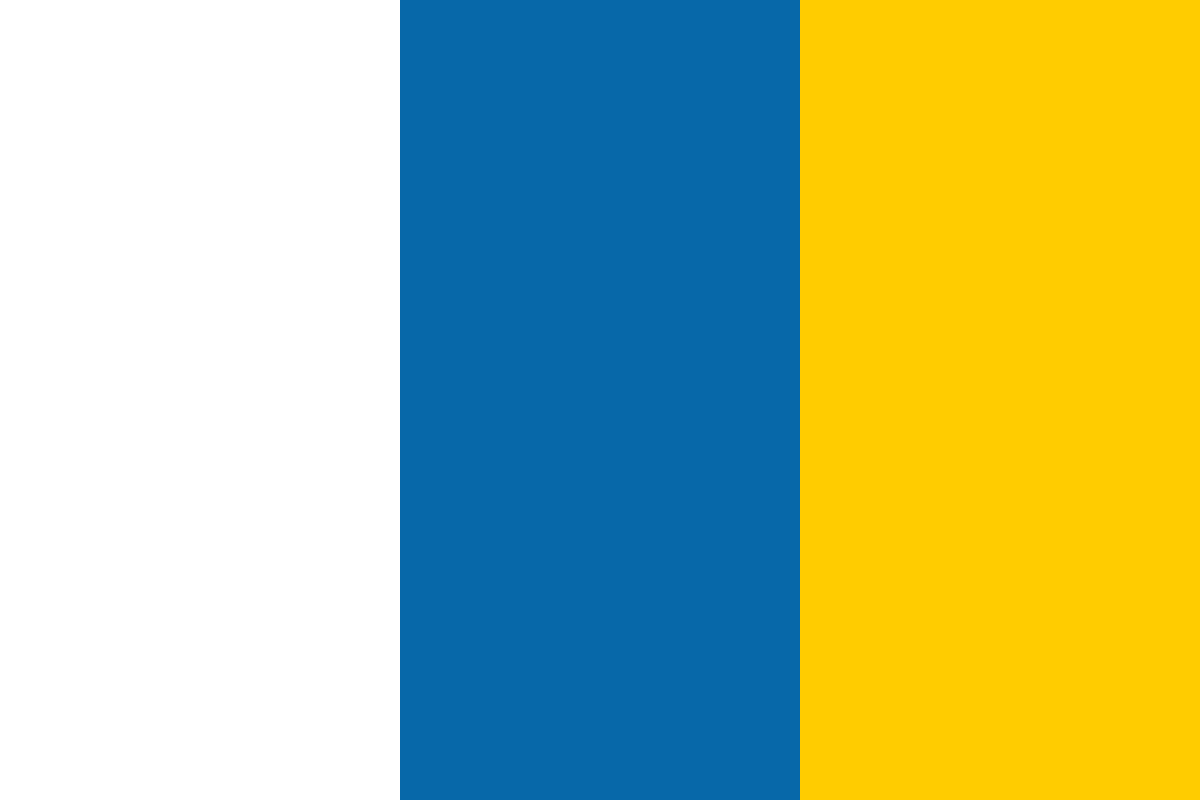

































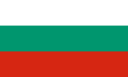








Before and during surgery anesthesiologists need to keep track of the anesthesia administered and the patient’s vitals. Imbalance in the level of anesthesia can cause low oxygen levels in the blood known as hypoxemia. Currently, there is no system to predict when this could happen during surgery and the patient is at the mercy of an anesthesiologist’s experience and discretion.
A team of researchers from the University of Washington have come up with a system to predict if a patient is at the risk of hypoxemia. This is done using patient data like age and body mass index. Data from 50,000 surgeries was collected to train the machine learning model.
The team wanted the model to solve two different kinds of problems.
While predicting, for the first problem, the BMI was a crucial factor while for the second, the oxygen levels. Then, Lee and Lundberg worked on a new approach to train Prescience in a way that it would generate understandable explanations behind its predictions.
Now it was time to test Prescience. Lee and Lundberg created a web interface. It ran the anesthesiologists through cases from surgeries in the dataset that were not used to train Prescience. For the real-time test, the researchers specifically chose cases that would be hard to predict. For example, when a patient’s blood oxygen level is stable for 10 minutes and then drops.
It was noted that Prescience improved the ability of doctors to correctly predict a patient’s hypoxemia risk by 16 percent before a surgery. In real-time, during a surgery it was able to predict the risk by 12 percent. With the help of Prescience, the anesthesiologists were able to correctly distinguish between the two scenarios nearly 80 percent of the time both before and during surgery.
Prescience is not ready to be used in real operations yet. Lee and Lundberg plan to continue working with anesthesiologists to improve Prescience. In addition to hypoxemia, the team hopes to predict low blood pressure and recommend appropriate treatment plans with Prescience in the future.
Although they could have successfully built a model that could predict hypoxemia, the researchers also wanted to answer the question “Why?”. A change from the traditional black box AI models engineers and researchers are used to.
Lee, author of the paper said: “Modern machine-learning methods often just spit out a prediction result. They don’t explain to you what patient features contributed to that prediction. Our new method opens this black box and actually enables us to understand why two different patients might develop hypoxemia. That’s the power.”
The research team consisted of four people, two from medicine and two from computer science. Bala Nair, research associate professor of anesthesiology and pain medicine at the UW School of Medicine, Su-In Lee, an associate professor in the UW’s Paul G. Allen School of Computer Science & Engineering, Monica Vavilala, professor of anesthesiology and pain medicine at the UW School of Medicine and Scott Lundberg, a doctoral student in the Allen School.
The system is not meant to replace doctors.
You can read the research paper at Nature science journal and the University of Washington website.
Swarm AI that enables swarms of radiologists, outperforms specialists or AI alone in predicting Pneumonia
How to predict viral content using random forest regression in Python [Tutorial]
SAP creates AI ethics guidelines and forms an advisory panel