










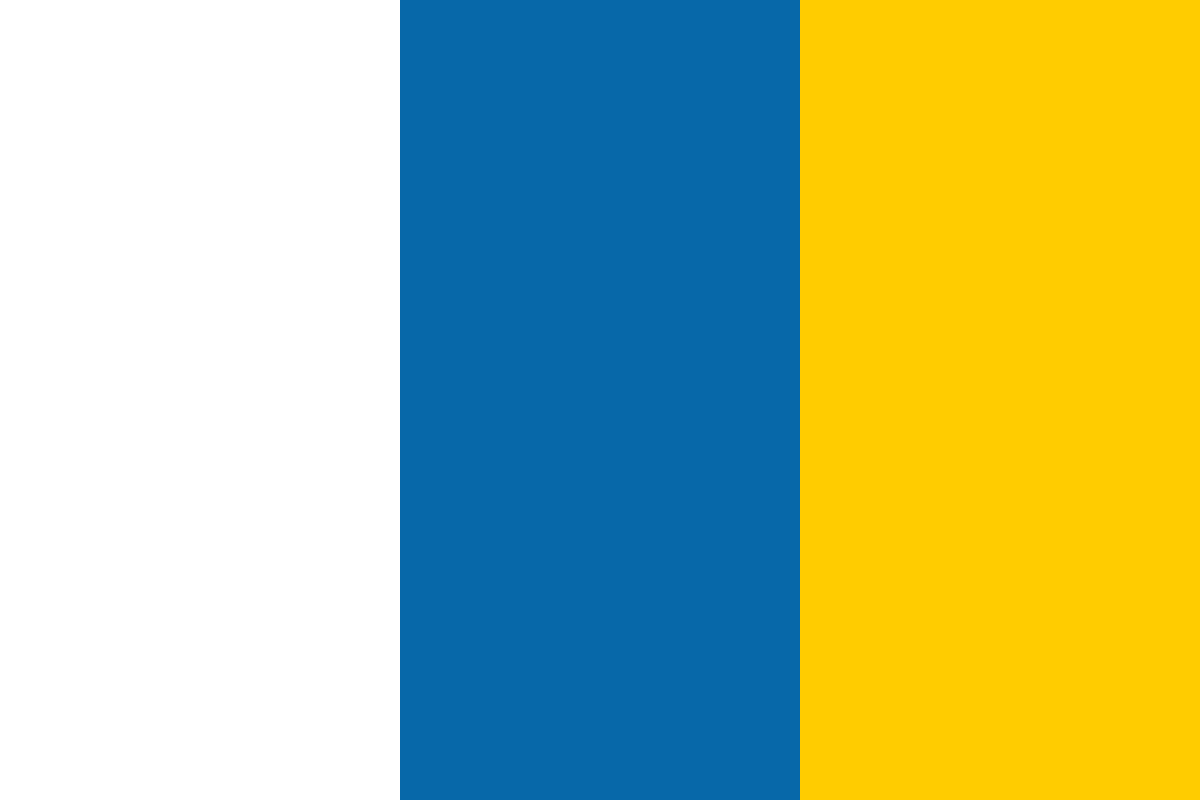

































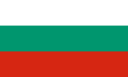








It was last year in May when Facebook AI Research (FAIR) released an open source ‘ELF’ OpenGo bot, an AI bot that has defeated world champion professional Go players, based onits existing ELF platform for Reinforcement Learning Research. Yesterday, FAIR announced new features and research results related to ELF OpenGo, including an updated model, a Windows executable version of the bot, and a unique archive analyzing 87k professional Go games.
ELF OpenGo, an open-source reimplementation of the AlphaZero algorithm, is the first open-source Go AI that has convincingly demonstrated superhuman performance, achieving a (20:0) record against global top professionals.
The FAIR team have updated the ELF OpenGo model, by re-training the model from scratch, the team has also provided a data set of 20 million self-play games and the 1,500 intermediate models used to generate them. This in turn, further reduces the need for computing resources.
The FAIR team further used the new model to analyze the games played by professional human players, however, they observed that its ability to predict the players’ moves went down very early during its learning process (after less than 10 percent of the total training time). But, as the model continued undergoing training, its skills levels also kept on improving. This ultimately led to it beating the team’s earlier prototype ELF OpenGo model 60 percent of the time.
“That prototype system was already outperforming human experts, having achieved a 20-0 record against four Go professionals ranked among the top 30 players in the world”, states the team. However, the exploration of ELF OpenGo's learning process also revealed some important limitations specific to deep RL. For instance, similar to AlphaZero, new ELF OpenGo model never fully master the concept of “ladders" i.e. a common technique in which one player traps the other's stones in a long formation. FAIR team then curated a data set of 100 ladder scenarios and evaluated ELF OpenGo's performance with them.
The team has come out with a new interactive tool based on ELF OpenGo's analysis of 87,000 games played by humans. This data set spans 1700 to 2018 and their system is constantly evaluating the quality of individual moves depending on the agreement between the moves predicted by the bot and the human players. “Though the tool encourages deep dives into specific matches, it also highlights important trends in Go. In analyzing games played over that period of more than 300 years, the bot found average strength of play has improved fairly steadily”, states the team. You can also analyze individual players such as Honinbo Shusaku, (the most famous Go player in history), which in turn would show different trends in comparison with ELF OpenGo, depending on the stage within the gameplay.
“Though ELF OpenGo is already being used by research teams and players around the world, we're excited to expand last year's release into a broader suite of open source resources. By making our tools and analysis fully available, we hope to accelerate the AI community's pursuit of answers to these questions”, states the FAIR team.
For more information, check out the official ELF OpenGo Bot announcement.
Facebook’s artificial intelligence research team, FAIR, turns five. But what are its biggest accomplishments?
Deepmind’s AlphaZero shows unprecedented growth in AI, masters 3 different games
Google DeepMind’s AI AlphaStar beats StarCraft II pros TLO and MaNa; wins 10-1 against the gamers