










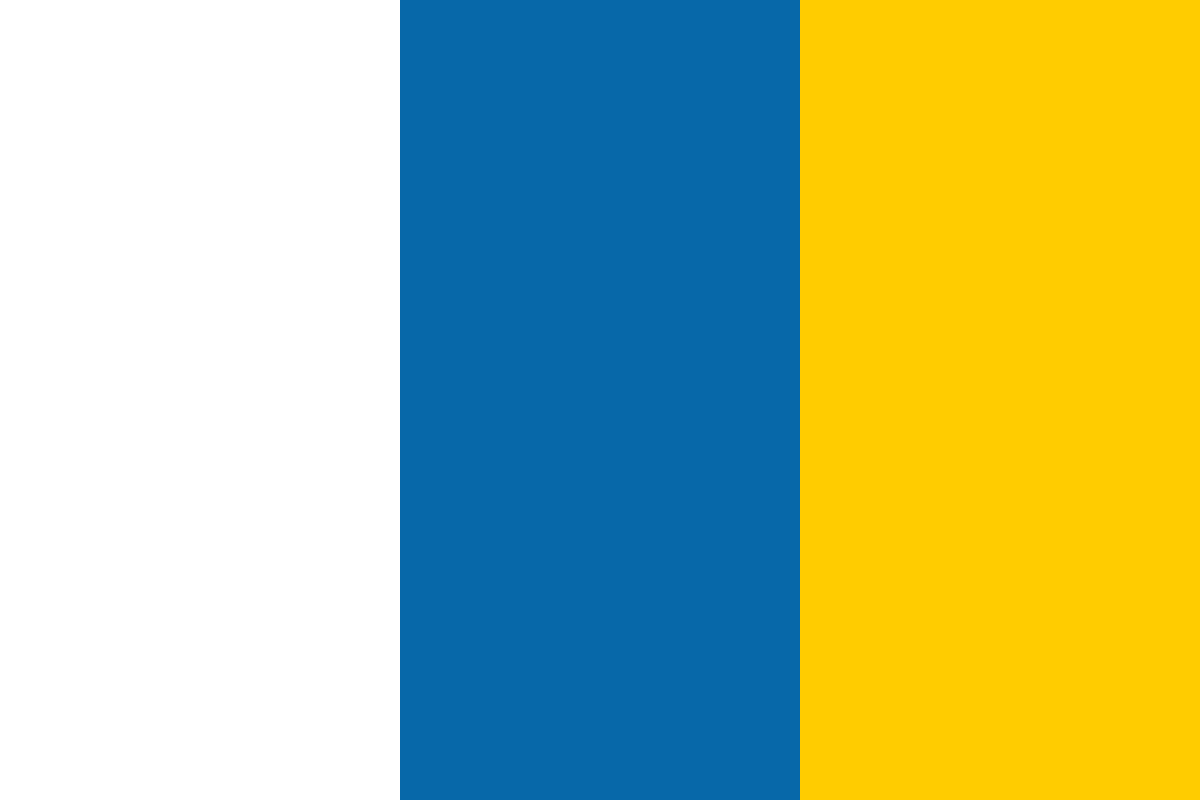

































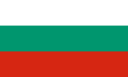








Games these days are closer to reality than ever. Life-like graphics, smart gameplay and realistic machine-human interactions have led to major game studios up the ante when it comes to adopting the latest and most up to date tech for developing games. In fact, not so long ago, we shared with you a few interesting ways in which Artificial Intelligence is transforming the gaming industry. Inclusion of deep learning in games has emerged as one popular solution to make the games smarter.
Deep learning can be used to enhance the realism and excitement in games by teaching the game agents how to behave more accurately, and in a more life-like manner. We recently came up with this interesting implementation of deep learning to to play the game of FIFA 18, and we were quite impressed!
Using just 2 layers of neural networks and with a limited amount of training, the bot that was developed managed to learn the basic rules of football (soccer). Not just that, it was also able to perform the basic movements and tasks in the game correctly. To achieve this, 2 neural networks were developed - a Convolutional Neural Network to detect objects within the game, and a second layer of LSTM (Long Short Term Memory) network to specify the movements accordingly.
The same user also managed to leverage deep learning to improve the in-game graphics of the FIFA 18 game. Using the deepfakes algorithm, he managed to swap the in-game face of one of the players with the player’s real-life face. The reason? The in-game faces, although quite realistic, could be better and more realistic. The experiment ended up being near perfect, as the resultant face that was created was as good as perfect. How did he do it? After gathering some training data which was basically some images of players scraped off Google, the user managed to train two autoencoders which learnt the distinction between the in-game face and the real-world face. Then, using the deepfakes algorithm, the inputs were reversed, recreating the real-world face in the game itself. The difference is quite astonishing:
Apart from improving the gameplay and the in-game character graphics, deep learning can also be used to enhance the way the opponents/adversaries interact with the player in the game. If we take the example of the FIFA game mentioned before, deep learning can be used to enhance the behaviour and appearance of the in-game crowd, who can react or cheer their team better as per their team’s performance.
The following are some of the clear advantages of implementing deep learning techniques in games:
Another obvious question comes to mind at this stage, however. What are the drawbacks of implementing deep learning for games? A few come to mind immediately:
With advancements in technology and better, faster hardware, many of the current limitations in developing smarter games can be overcome. Fast generative models can look into the real-time generation of images, while faster graphic cards can take care of the model computation issue. All in all, dabbling with deep learning in games seems worth the punt which game studios should definitely think of taking.
What do you think? Is incorporating deep learning techniques in games a scalable idea?