










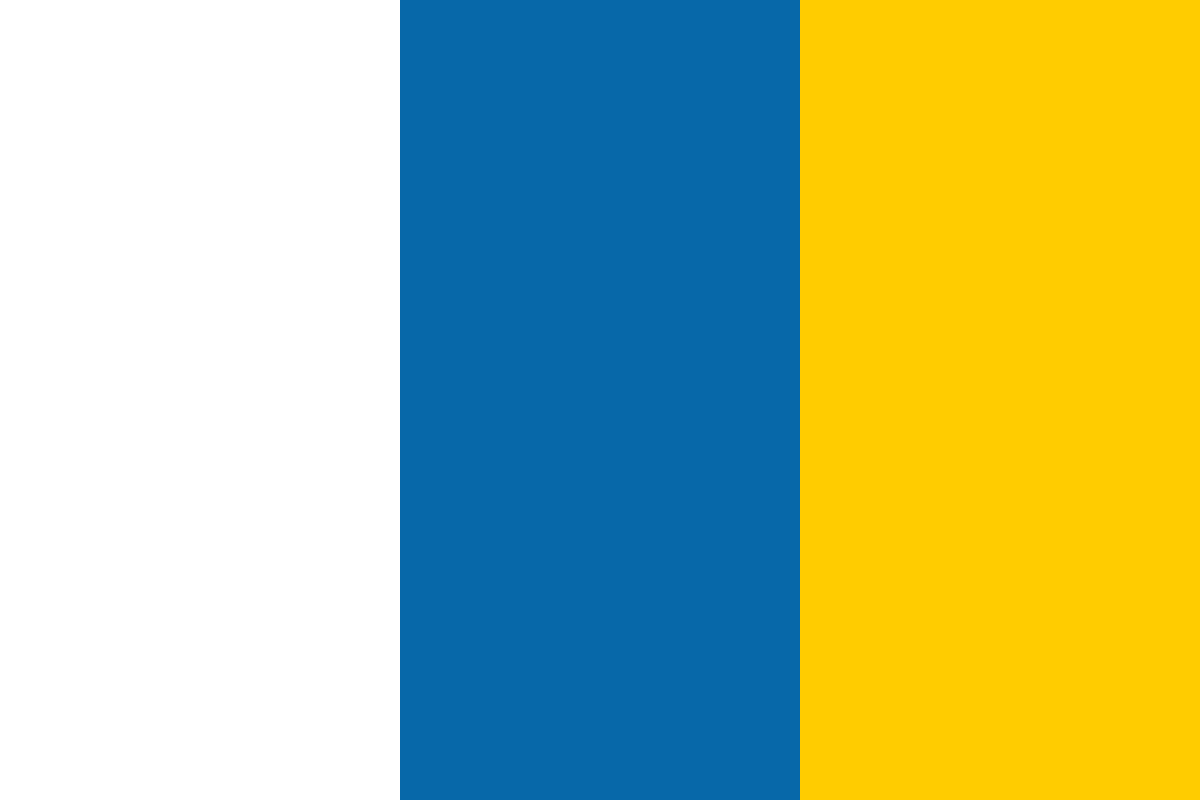

































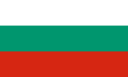








LinkedIn is used by 567M users every day. It creates new opportunities for connecting with professionals all over the globe, with more than a million posts, videos, and articles made on the LinkedIn feed each day.
However, the team has identified that the growth in the number of post creators and viewers have led to issues. These include almost no recognition for lesser-known creators and viral posts drowning out posts from closer connections
To combat this, the team combined multiple experimental techniques and came up with a smarter feed relevance model.
The team discovered that there was no equal distribution of reactions given by members on a creator’s post. In other words, the number of creators who get zero feedback on making a post was actually increasing. This posed a huge problem as getting feedback is a motivational boost for creators to continue posting in the future.
Influencers with millions of followers do get more reactions as compared to the average person. If feed viewers kept giving feedback to the top 1% of posters, who were already getting plenty of attention, the lesser-known creators would not be recognized at all.
A second issue encountered was that the team received anecdotal reports pointing out that irrelevant hyper-viral posts were gaming the feed and crowding out posts from closer connections.
The original LinkedIn feed model was designed in a way that if many people have already enjoyed, liked, and shared a piece of content, then the feed will correctly guess that a new viewer is also highly likely to enjoy it and hence show highly viral content. This meant viewers missed important posts from close connections and people they know personally. Moreover, the model was not programmed to consider how much the creator may appreciate receiving feedback from the viewer.
The solution: A new Optimization function
The team added an additional term in the optimization function of the relevance model. Once a creator receives feedback from the viewer, the term quantifies the value received. Now that the feed knew how much a given creator will appreciate getting feedback from a given viewer, it uses this information to rank posts. The model also takes into account ‘spam feedback’ considering the quality of the post to avoid spamming viewers with low-quality posts. This consideration for small creators ensures that no one is left behind and they can easily reach out to the community.
Optimization from the creator’s perspective
To test the model, the team performed an “upstream/downstream metrics.”
They used a collection of upstream metrics, for example: “first likes given.” This metric quantifies how often a feed viewer likes a post that didn’t previously have any likes. If the viewer sees a post that doesn’t have any likes, and he clicks the like button for it, then it creates the test case of “first like given.” The other metric used was called “creator love” that describe how the creator feels about the viewer’s actions. It also decides the impact that the viewers’ actions has on a creators post.
“creator love” upstream metrics
The suite of metrics contains several variations on test cases involving comments, freshness of the post, and the changing value of feedback beyond the first piece. It all boils down to measuring a value given to the creator.
The team also used edge-based bootstrapping on bernoulli randomization, pioneered by Jim Sorenson.
The answer is 'yes!'. This feature turned out to be successful for both creators and feed viewers. The team believes this change is helping posts from close connections to appears at the top of a member’s feed. And members like seeing more content from people they know! This model especially benefited creators with smaller networks. The model was supposed to take about 8% of feedback away from the top 0.1% of creators and redistribute it to the bottom 98%. This worked and showed a 5% increase in creators returning to post again. As for top creators, taking 8% of the likes away from the top 0.1% still leaves them better off than they were a year ago. These changes just help to ensure equality among all members of the network.
It will interesting to see the impact that this new model will have on viewers feed and their reaction to the same. To more in-depth about the experiments the team performed on the model and their line of thoughts, you can visit their official blog.
What is Statistical Analysis and why does it matter?
Working with Azure container service cluster [Tutorial]
Performing Sentiment Analysis with R on Obama’s State of the Union speeches [Tutorial]