










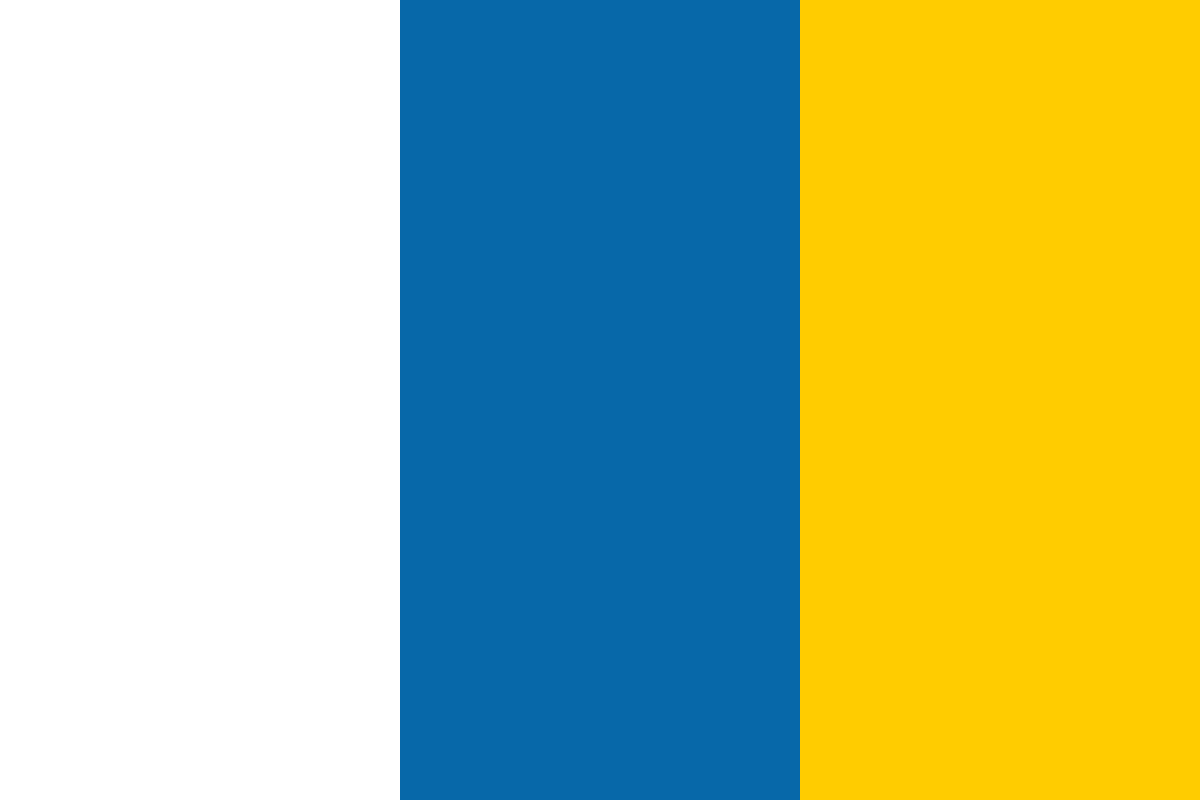

































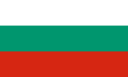








This year’s Skill Up survey threw a spotlight on the challenges developers and engineering teams face when it comes to cloud. Indeed, it even highlighted the extent to which cloud is still a nascent trend for many developers, even though it feels so mainstream within the industry - almost half of respondents aren’t using cloud at all.
But for those that do use cloud, the survey results also illustrated some of the specific ways that people are using or plan to use cloud platforms, as well as highlighting the biggest challenges and mistakes organisations are making when it comes to cloud.
What came out as particularly important is that the limitations and the opportunities of cloud must be thought of together. With our research finding that cost only becomes important once a cloud platform is being used, it’s clear that if we’re to successfully - and cost effectively - use the cloud platforms we do, understanding the relationship with cost and opportunity over a sustained period of time (rather than, say, a month) is absolutely essential. As one of our respondents told us “businesses are still figuring out how to leverage cloud computing for their business needs and haven't quite got the cost model figured out.”
In this year’s survey, we asked people what their primary motivations for using cloud are. The key motivators were use case and employment (ie. the decision was out of the respondent’s hands), but it was striking to see cost as only a minor consideration. Placed in the broader context of discussions around efficiency and a tightening global market, this seemed remarkable. It appears that people aren’t entering the cloud marketplace with cost as a top consideration.
In contrast however, this picture changes when we asked respondents about the biggest limiting factors for their chosen cloud platforms. At this point, cost becomes a much more important factor.
This highlights that the reality of cloud costs only become apparent - or rather, becomes more apparent - once a cloud platform is implemented and being used. From this we can infer that there is a lack of strategic planning in cloud purchasing. It’s almost as if technology leaders are falling into certain cloud platforms based on commonplace assumptions about what’s right.
This then has consequences further down the line.
The fact that functionality is also a key limitation is also important to note here - in fact, it is actually closely tied up with cost, insofar as the functionality of each respective cloud platform is very neatly defined by its pricing structure. Take serverless, for example - although it’s typically regarded as something that can be cost-effective for organizations, it can prove costly when you start to scale workloads. You might save more money simply by optimizing your infrastructure.
What this means in practice is that the features you want to exploit within your cloud platform should be approached with a clear sense of how it’s going to be used and how it’s going to fit in the evolution of your business and technology in the medium and long term future.
There were two distinct trends that developers identified as the most exciting: machine learning and serverless.
Although both are very different, they both hold a promise of efficiency. Whether that’s the efficiency in moving away from traditional means of hosting to cloud-based functions to powerful data processing and machine-led decision making at scale, the fundamentals of both trends are about managing economies of scale in ways that would have been impossible half a decade ago.
This plays into some of the issues around cost. If serverless and machine learning both appear to offer ways of saving on spending or radically driving growth, when that doesn’t quite turn out in the way technology purchasers expected it would, the relationship between cost and features can become a little bit strained.
The idea that serverless will save you money is popular. And in general, it is inexpensive. The pricing structures of both AWS and Azure make Functions as a Service (FaaS) particularly attractive. It means you’ll no longer be spending money on provisioning compute resources you don’t actually need, with your provider managing the necessary elasticity.
Read next: The Future of Cloud lies in revisiting the designs and limitations of today’s notion of ‘serverless computing’, say UC Berkeley researchers
However, as we've already seen, serverless doesn't guarantee cost efficiency. You need to properly understand how you're going to use serverless to ensure that it's not costing you big money without you realising it.
One way of using it might be to employ it for very specific workloads, allowing you to experiment in a relatively risk-free manner before employing it elsewhere - whatever you decide, you must ensure that the scope and purpose of the project is clear.
Machine learning - or deep learning in particular - is very expensive to do. This is one of the reasons that machine learning on cloud - machine learning as a service - is one of the most attractive features of many cloud platforms.
But it’s not just about cost. Using cloud-based machine learning tools also removes some of the barriers to entry, making it easier for engineers who don’t necessarily have extensive training in the field to actually start using machine learning models in various ways.
However, this does come with some limitations - and just as with serverless, you really do need to understand and even visualize how you’re going to use machine learning to ensure that you’re not just wasting time and energy with machine learning cloud features.
You need to be clear about exactly how you’re going to use machine learning, what data you’re going to use, where it’s going to be stored, and what the end result should look like. Perhaps you want to embed machine learning capabilities inside an app? Or perhaps you want to run algorithms on existing data to inform internal decisions? Whatever it is, all these questions are important.
These types of questions will also impact the type of platform you select. Google’s Cloud Platform is far and away the go-to platform for machine learning (this is one of the reasons why so many respondents said their motivation for using it was use case), but bear in mind that this could lead to some issues if the bulk of your data is typically stored on, say, AWS - you’ll need to build some kind of integration, or move your data to GCP (which is always going to be a headache).
These types of extras are really important to consider when it comes to leveraging exciting cloud features. Yes you need to use a pricing calculator and spend time comparing platforms, but factoring additional development time to build integrations or move things is something that a calculator clearly can’t account for.
Indeed, this is true in the context of both machine learning and serverless. The organizational implications of your purchases are perhaps the most important consideration and one that’s often the easiest to miss.
However, although the organizational implications aren’t necessarily problems to be resolved - they could well be opportunities that you need to embrace. You need to prepare and be ready for those changes.
Ultimately, preparation is key when it comes to leveraging the benefits of cloud. Defining the scope is critical and to do that you need to understand what your needs are and where you want to get to. That sounds obvious, but it’s all too easy to fall into the trap of focusing on the possibilities and opportunities of cloud without paying careful consideration to how to ensure it works for you.
Read the results of Skill Up 2019. Download the report here.