










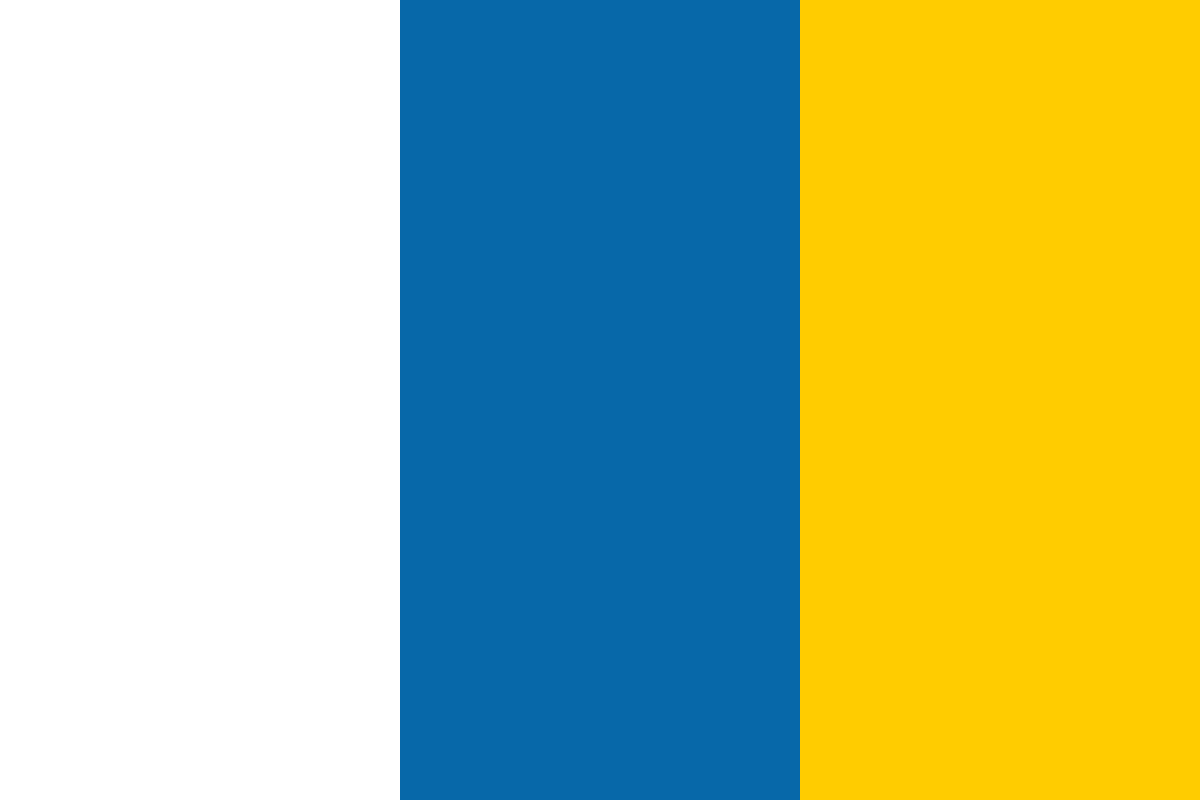

































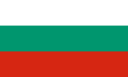








Many moving parts have to be tied together for an ML model to execute and produce results successfully. This process of tying together different pieces of the ML process is known as pipelines. A pipeline is a generalized concept but a very important concept for a Data Scientist. In software engineering, people build pipelines to develop software that is exercised from source code to deployment. Similarly, in ML, a pipeline is created to allow data flow from its raw format to some useful information. It provides a mechanism to construct a multi-ML parallel pipeline system in order to compare the results of several ML methods.
In this tutorial, we see how to create our own AutoML pipelines. You will understand how to build pipelines in order to handle the model building process.
Each stage of a pipeline is fed processed data from its preceding stage; that is, the output of a processing unit is supplied as an input to its next step. The data flows through the pipeline just as water flows in a pipe. Mastering the pipeline concept is a powerful way to create error-free ML models, and pipelines form a crucial element for building an AutoML system.
The code files for this article are available on Github.
This article is an excerpt from a book written by Sibanjan Das, Umit Mert Cakmak titled Hands-On Automated Machine Learning.
Usually, an ML algorithm needs clean data to detect some patterns in the data and make predictions over a new dataset. However, in real-world applications, the data is often not ready to be directly fed into an ML algorithm. Similarly, the output from an ML model is just numbers or characters that need to be processed for performing some actions in the real world. To accomplish that, the ML model has to be deployed in a production environment. This entire framework of converting raw data to usable information is performed using a ML pipeline.
The following is a high-level illustration of an ML pipeline:
We will break down the blocks illustrated in the preceding figure as follows:
As we see, there are several stages that we will need to perform to get results out of an ML model. The scikit-learn provides us a pipeline functionality that can be used to create several complex pipelines. While building an AutoML system, pipelines are going to be very complex, as many different scenarios have to be captured. However, if we know how to preprocess the data, utilizing an ML algorithm and applying various evaluation metrics, a pipeline is a matter of giving a shape to those pieces.
Let's design a very simple pipeline using scikit-learn.
We will first import a dataset known as Iris, which is already available in scikit-learn's sample dataset library (http://scikit-learn.org/stable/auto_examples/datasets/plot_iris_dataset.html). The dataset consists of four features and has 150 rows. We will be developing the following steps in a pipeline to train our model using the Iris dataset. The problem statement is to predict the species of an Iris data using four different features:
In this pipeline, we will use a MinMaxScaler method to scale the input data and logistic regression to predict the species of the Iris. The model will then be evaluated based on the accuracy measure:
from sklearn.datasets import load_iris from sklearn.preprocessing import MinMaxScaler from sklearn.linear_model import LogisticRegression from sklearn.model_selection import train_test_split from sklearn.pipeline import Pipeline
# Load and split the data iris = load_iris() X_train, X_test, y_train, y_test = train_test_split(iris.data, iris.target, test_size=0.2, random_state=42) X_train.shape
print(X_train)
The preceding code provides the following output:
pipe_lr = Pipeline([('minmax', MinMaxScaler()), ('lr', LogisticRegression(random_state=42))])
pipe_lr.fit(X_train, y_train)
score = pipe_lr.score(X_test, y_test) print('Logistic Regression pipeline test accuracy: %.3f' % score)
As we can note from the following results, the accuracy of the model was 0.900, which is 90%:
In the preceding example, we created a pipeline, which constituted of two steps, that is, minmax scaling and LogisticRegression. When we executed the fit method on the pipe_lr pipeline, the MinMaxScaler performed a fit and transform method on the input data, and it was passed on to the estimator, which is a logistic regression model. These intermediate steps in a pipeline are known as transformers, and the last step is an estimator.
Transformers are used for data preprocessing and has two methods, fit and transform. The fit method is used to find parameters from the training data, and the transform method is used to apply the data preprocessing techniques to the dataset.
Estimators are used for creating machine learning model and has two methods, fit and predict. The fit method is used to train a ML model, and the predict method is used to apply the trained model on a test or new dataset.
This concept is summarized in the following figure:
We have to call only the pipeline's fit method to train a model and call the predict method to create predictions. Rest all functions that is, Fit and Transform are encapsulated in the pipeline's functionality and executed as shown in the preceding figure.
Sometimes, we will need to write some custom functions to perform custom transformations. The following section is about function transformer that can assist us in implementing this custom functionality.
A FunctionTransformer is used to define a user-defined function that consumes the data from the pipeline and returns the result of this function to the next stage of the pipeline. This is used for stateless transformations, such as taking the square or log of numbers, defining custom scaling functions, and so on.
In the following example, we will build a pipeline using the CustomLog function and the predefined preprocessing method StandardScaler:
import numpy as np from sklearn.datasets import load_iris from sklearn.model_selection import train_test_split from sklearn import preprocessing from sklearn.pipeline import make_pipeline from sklearn.preprocessing import FunctionTransformer from sklearn.preprocessing import StandardScaler
def CustomLog(X): return np.log(X)
def PreprocData(X, Y): pipe = make_pipeline( FunctionTransformer(CustomLog),StandardScaler() ) X_train, X_test, Y_train, Y_test = train_test_split(X, Y) pipe.fit(X_train, Y_train) return pipe.transform(X_test), Y_test
iris = load_iris() X, Y = iris.data, iris.target print(X)
The preceding code prints the following output:
X_transformed, Y_transformed = PreprocData(X, Y) print(X_transformed)
We will now need to build various complex pipelines for an AutoML system. In the following section, we will create a sophisticated pipeline using several data preprocessing steps and ML algorithms.
In this section, we will determine the best classifier to predict the species of an Iris flower using its four different features. We will use a combination of four different data preprocessing techniques along with four different ML algorithms for the task. The following is the pipeline design for the job:
We will proceed as follows:
from sklearn.datasets import load_iris from sklearn.preprocessing import StandardScaler from sklearn.decomposition import PCA from sklearn.preprocessing import MinMaxScaler from sklearn.model_selection import train_test_split from sklearn.neighbors import KNeighborsClassifier from sklearn.ensemble import RandomForestClassifier from sklearn import svm from sklearn import tree from sklearn.pipeline import Pipeline
# Load and split the data iris = load_iris() X_train, X_test, y_train, y_test = train_test_split(iris.data, iris.target, test_size=0.2, random_state=42)
The following is the code snippet for creating these four different pipelines used to create four different models:
# Construct svm pipeline
pipe_svm = Pipeline([('ss1', StandardScaler()),
('pca', PCA(n_components=2)),
('svm', svm.SVC(random_state=42))])
# Construct knn pipeline
pipe_knn = Pipeline([('ss2', StandardScaler()),
('knn', KNeighborsClassifier(n_neighbors=6, metric='euclidean'))])
# Construct DT pipeline
pipe_dt = Pipeline([('ss3', StandardScaler()),
('minmax', MinMaxScaler()),
('dt', tree.DecisionTreeClassifier(random_state=42))])
# Construct Random Forest pipeline
num_trees = 100
max_features = 1
pipe_rf = Pipeline([('ss4', StandardScaler()),
('pca', PCA(n_components=2)),
('rf', RandomForestClassifier(n_estimators=num_trees, max_features=max_features))])
pipe_dic = {0: 'K Nearest Neighbours', 1: 'Decision Tree', 2:'Random Forest', 3:'Support Vector Machines'}
pipelines = [pipe_knn, pipe_dt,pipe_rf,pipe_svm]
In the following code snippet, we fit each of the four pipelines iteratively to the training dataset:
# Fit the pipelines for pipe in pipelines: pipe.fit(X_train, y_train)
# Compare accuracies for idx, val in enumerate(pipelines): print('%s pipeline test accuracy: %.3f' % (pipe_dic[idx], val.score(X_test, y_test)))
best_accuracy = 0 best_classifier = 0 best_pipeline = '' for idx, val in enumerate(pipelines): if val.score(X_test, y_test) > best_accuracy: best_accuracy = val.score(X_test, y_test) best_pipeline = val best_classifier = idx print('%s Classifier has the best accuracy of %.2f' % (pipe_dic[best_classifier],best_accuracy))
To summarize, we learned about building pipelines for ML systems. The concepts that we described in this article gave you a foundation for creating pipelines.
To have a clearer understanding of the different aspects of Automated Machine Learning, and how to incorporate automation tasks using practical datasets, do checkout the book Hands-On Automated Machine Learning.