










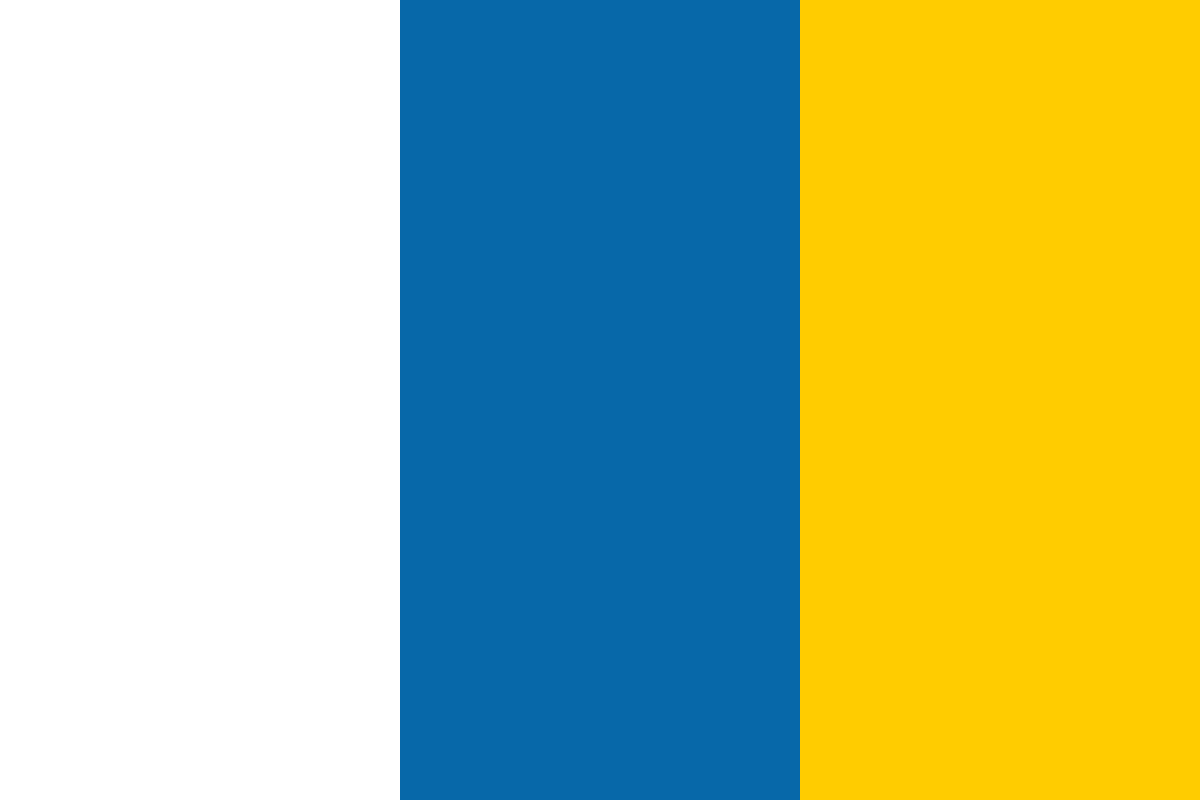

































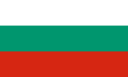








We know your time is valuable. That's why what matters is important. We've written about the trends and issues that are going to matter in data science, but here you can find 5 data science tools that you need to pay attention to in 2018.
Read our 5 things that matter in data science in 2018 here.
Google's TensorFlow has been one of the biggest hits of 2017 when it comes to libraries. It’s arguably done a lot to make machine learning more accessible than ever before. That means more people actually building machine learning and deep learning algorithms, and the technology moving beyond the domain of data professionals and into other fields. So, if TensorFlow has passed you by we recommend you spend some time exploring it. It might just give your skill set the boost you’re looking for.
Explore TensorFlow content here.
Jupyter isn’t a new tool, sure. But it’s so crucial to the way data science is done that it’s importance can’t be understated. And as pressure is placed on data scientists and analysts to communicate and share data in ways that empower stakeholders in a diverse range of roles and departments. It’s also worth mentioning its relationship with Python - we’ve seen Python go from strength to strength throughout 2017, and showing no signs of letting up; the close relationship between the two will only serve to make Jupyter more popular across the data science world.
Discover Jupyter eBooks and videos here.
In a year when deep learning has captured the imagination, it makes sense to include both libraries helping to power it. It’s a close call between Keras and TensorFlow which deep learning framework is ‘better’ - ultimately, like everything, it’s about what you’re trying to do. This post explores the difference between Keras and TensorFlow very well - the conclusion is ultimately that while TensorFlow offers more ‘control’, Keras is the library you want if you simply need to get up and running. Both libraries have had a huge impact in 2017, and we’re only going to be seeing more of them in 2018.
Learn Keras. Read Deep Learning with Keras.
Automated machine learning is going to become incredibly important in 2018. As pressure mounts on engineers and analysts to do more with less, tools like Auto SKLearn will be vital in reducing some of the ‘manual labour’ of algorithm selection and tuning.
This one might be a little unexpected. We know just how popular Apache Spark is when it comes to distributed and parallel computing, but Dask represents an interesting competitor that’s worth watching throughout 2018. It’s high-level API integrates exceptionally well with Python libraries like NumPy and pandas; it’s also much more lightweight than Spark, so it could be a good option if you want to avoid building out a weighty big data tech stack.
Explore Dask in the latest edition of Python High Performance.