










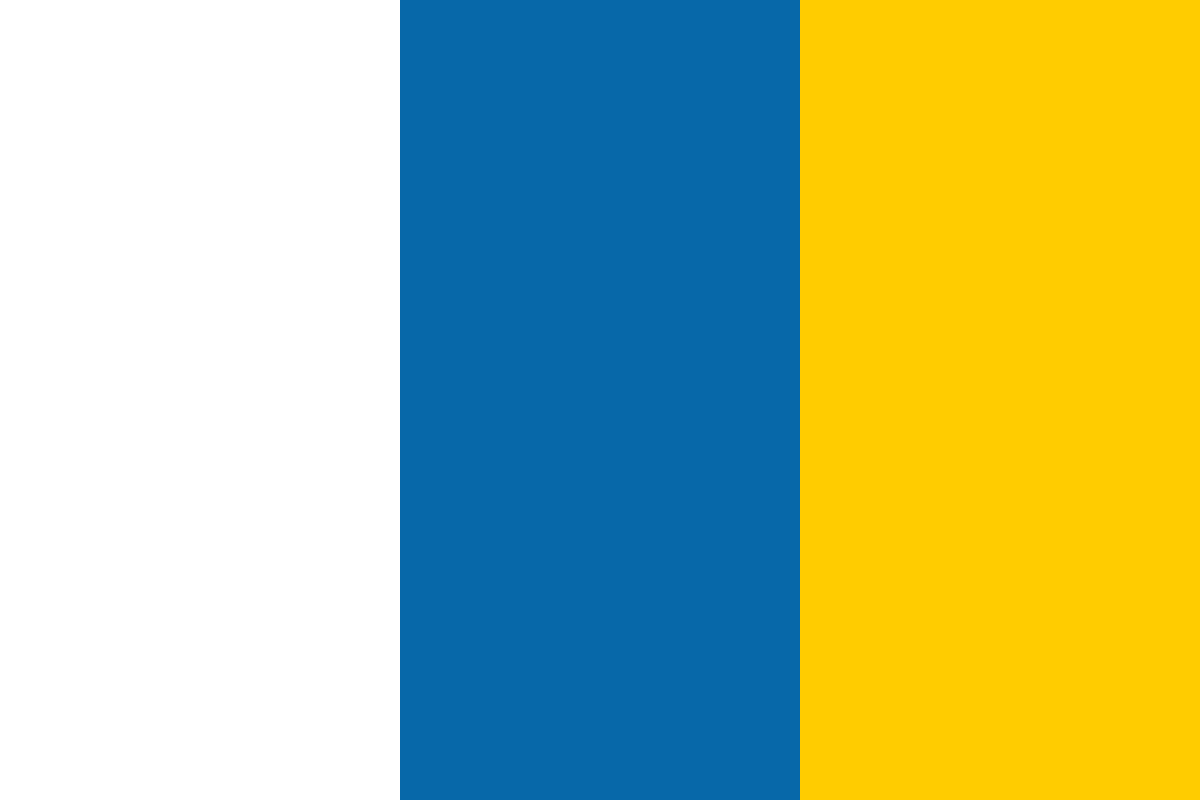

































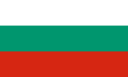








Fortunately, machine learning can help support a more robust, reliable and efficient security initiative. Here are just 4 ways machine learning can support your software security strategy.
We have seen in the past how a single gap in endpoint protection resulted in serious data breaches. In May this year, Mexican fast food giant Chipotle learned the hard way when cybercriminals exploited the company's point of sale systems to steal credit card information. The Chiptole incident was a very real reminder for many retailers to patch critical endpoints on a regular basis. It is crucial to guard your company’s endpoints which are virtual front doors to your organization’s precious information. Your cybersecurity strategy must consider a holistic endpoint protection strategy to secure against a variety of threats, both known and unknown.
Traditional endpoint security approaches are proving to be ineffective and costing businesses millions in terms of poor detection and wasted time. The changing landscape of the cybersecurity market brings with it its own set of unique challenges (Palo Alto Networks have highlighted some of these challenges in their whitepaper here). Sophisticated Machine Learning techniques can help fight back threats that aren’t easy to defend with traditional ways. One could achieve this by adopting any of the three ML approaches: Supervised machine learning, unsupervised machine learning and reinforcement learning. Establishing the right machine learning approach entails a significant understanding of your expectations from the endpoint protection product. You might consider checking on the speed, accuracy, and efficiency of the machine learning based endpoint protection solution with the vendor to make an informed choice of what you are opting for.
We recommend the use of a supervised machine learning approach for endpoint protection as it’s a proven way of malware detection and it delivers accurate results. The only catch is that these algorithms require relevant data in sufficient quantity to work on and the training rounds need to be speedy and effective to guarantee efficient malware detection. Some of the popular ML-based endpoint protection options available in the market are Symantec Endpoint Protection 14, CrowdStrike, and TrendMicro’s XGen.
Predictive analytics is no longer just restricted to data science. By adopting predictive analytics, you can take a proactive approach to cybersecurity too. Predictive analytics makes it possible to not only identify infections and threats after they have caused damage, but also to raise an alarm for any future incidents or attacks. Predictive analytics is a crucial part of the learning process for the system. With sophisticated detection techniques the system can monitor network activities and report real-time data.
One incredibly effective technique organizations are now beginning to use is a combination of advanced predictive analytics with a red team approach. This enables organizations to think like the enemy and model a broad range of threats. This process mines and captures large sets of data which is then processed. The real value here is the ability to generate meaningful insights out of the large data set collected and then letting the red team work on processing and identifying potential threats. This is then used by the organization to evaluate its capabilities, to prepare for future threats and to mitigate potential risks.
Behavior analytics is a highly trending area today in the cybersecurity space. Traditional systems such as antiviruses are skilled in identifying attacks based on historical data and matching signatures. Behavior analytics, on the other hand, detects anomalies and makes a judgement against what would be considered normal behaviour. As such, behavior analytics in enterprises is proving very effective when it comes to detecting intrusions that otherwise evade firewalls or antivirus software. It complements existing security measures such as firewall and antivirus rather than replacing them.
Behavior analytics work well within private cloud and infrastructures and is able to detect threats within internal networks. One popular example is Enterprise Immune System, by the vendor Darktrace, which uses machine learning to detect abnormal behavior in the system. It helps IT staff narrow down their perimeter of search and look out for specific security events through a visual console. What’s really promising is that because Darktrace uses machine learning, the system is not just learning from events within internal systems, but from events happening globally as well.
Trying to manage large amounts of data and logs generated from millions of IoT devices manually could be overwhelming if your company relies on the Internet of Things. Many a time, IoT devices are directly connected to the network which means it is fairly easy for attackers and hackers to take advantage of your inadequately protected networks. It could therefore be next to impossible to build a secure IoT system, if you set out to identify and fix vulnerabilities manually.
Machine learning can help you analyze and make sense of millions of data logs generated from IoT capable devices. Machine learning powered cybersecurity systems placed and seated directly inside your system can learn about security events as they happen. It can then monitor both incoming and outgoing IoT traffic in devices connected to the network and generate profiles for appropriate and inappropriate behavior inside your IoT ecosystem. This way the security system is able to react to even the slightest of irregularities and detect anomalies that were not experienced before. Currently, only a handful number of software and tools use Machine Learning or Artificial Intelligence for IoT security. But we are already seeing development on this front by major security vendors such as Symantec.
Surveys carried out frequently on IoT continue to highlight security as a major barrier to IoT adoption and we are hopeful that Machine Learning will come to the rescue.
Cyber crimes are evolving at a breakneck speed while businesses remain slow in adapting their IT security strategies to keep up with the times. Machine learning can help businesses make that leap to proactively address cyber threats and attacks by:
And, that’s just the beginning.
Have you used machine learning in your organization to enhance cybersecurity? Share with us your best practices and tips for using machine learning in cybersecurity in the comments below!